Introduction
The liver is the central organ in glucose, lipid, protein, and vitamin metabolism and produces coagulation factors and serum proteins (albumin, C-reactive protein (CRP)) [1]. Based on this variety of important functions for the whole organism, liver damage may affect other organ systems. The most prominent and most common example of this is non-alcoholic fatty liver disease (NAFLD), which is the liver manifestation of metabolic syndrome (MS) [2]. Non-alcoholic fatty liver disease is caused by excess lipid accumulation, which may harm the liver without apparent serological signs of injury [3]. Non-alcoholic fatty liver disease represents an emerging public health problem worldwide and is a major cause of liver-related morbidity and mortality [4, 5]. Non-alcoholic fatty liver disease is considered a multisystem disease [6], backed up by the fact that the most common cause of mortality in NAFLD is cardiovascular mortality [7, 8]. Evidence has accumulated suggesting a strong association between NAFLD and cardiovascular disease (CVD), for example different manifestations of subclinical atherosclerosis such as increased carotid intima-media thickness and carotid atherosclerosis [9, 10], endothelial dysfunction [11], arterial stiffness [12], impaired left ventricular function [13], and coronary calcification [9, 14]. In addition, increased serum levels of common markers of liver injury (γ-glutamyltransferase (GGT), alanine aminotransferase (ALT), aspartate aminotransferase (AST), alkaline phosphatase (AP), and bilirubin) are associated with greater risk of CVD events [15–18]. Recently, we demonstrated a link between liver enzymes and the severity of stenosis in acute myocardial infarction (AMI) [19]. Serum liver markers were relevant factors to identify high-risk subjects in a non-invasive prognostic score. There is also emerging evidence that NAFLD is associated with increased prevalence and incidence of chronic kidney disease (CKD), independently of multiple cardio-renal risk factors [20, 21].
The prognosis for patients after an AMI depends on several factors. Known factors include the extent of myocardium undergoing irreversible injury [22] and the type of the AMI (ST-elevation myocardial infarction (STEMI) versus non-ST-elevation myocardial infarction (NSTEMI)) [23], the time point of reperfusion [24], and the type of the implanted stent [23]. Age [25], gender [26], and cardiovascular risk factors are further essential factors which affect AMI patients’ outcome. However, it is currently unknown whether liver injury in early stages represented by liver enzymes is also associated with the long-term prognosis of patients after AMI or whether other non-invasive factors may have predictive properties.
In this retrospective study, data of patients after AMI were collected and analyzed for the potential predictive value of unbiased non-invasive parameters, including liver serum markers, for the long-term prognosis of patients after AMI. Demographic data and classic serum parameters for heart, renal, and liver diseases were analyzed for correlations with death.
Material and methods
Ethics statement
The study protocol conformed to the ethical guidelines of the 1975 Declaration of Helsinki and was approved by the Institutional Review Board (IRB; Ethik-Kommission der Medizinischen Fakultät der Universität Duisburg-Essen; Germany; reference number 15-6356-BO). Due to the retrospective nature of the study the IRB waived the need for written informed consent. All procedures adhered to the Declaration of Helsinki and the requirements of the IRB.
Study design and sample acquisition
In a retrospective single-center study, a cohort of 1242 patients was recruited. All patients had an AMI and underwent coronary angiography in the catheterization laboratory of the West German Heart and Vascular Centre Essen, University Hospital Essen. Acute myocardial infarction was defined as a troponin value above the 99th percentile of the upper reference level and either with ST-segment elevation or new left bundle-branch block on the electrocardiogram (STEMI, n = 117) [27], or without ST-segment elevation or new left bundle-branch block on the electrocardiogram (NSTEMI, n = 452) [28]. Patients were classified in two groups, non-survivors (n = 156) and survivors (n = 413), according to the follow-up of up to 6 years. Blood sampling was done directly before coronary intervention and serum parameters were determined in the central laboratory unit of the University Hospital Essen (Department of Clinical Chemistry and Laboratory Medicine) by standardized methods. Exclusion criteria were high-grade aortic valve disease, cardiomyopathy, cardiac allograft vasculopathy, endocarditis, hypertensive emergency, myocarditis, pericarditis, tachyarrhythmia absoluta by atrial fibrillation (atrial fibrillation > 100 bpm/min), and survival of sudden cardiac death. In addition, patients with a coronary artery disease after saphenous vein aortocoronary bypass grafting (SVG surgery) were excluded from the study. Patients with non-cardiac causes of troponin elevation were excluded: acute neurological disease including stroke or cerebral hemorrhage, acute pulmonary embolism, aortic dissection, diseases such as amyloidosis, sarcoidosis, or scleroderma, inflammatory myopathies such as polymyositis or dermatomyositis, sepsis, and patients who took cardio-toxic drugs (doxorubicin, 5-fluorouracil, trastuzumab). Finally, all patients for whom no follow-up was available were excluded (Figure 1, Flow diagram for patient selection), resulting in 569 eligible patients enrolled in the study.
Outcome, definitions and clinical follow-up
The main outcome of the study was cardiac death after 6 years. Secondary outcomes were major adverse cardiac events (MACE) at 6 years after the index procedure. The MACE was defined as one of the following conditions: AMI, cardiac death, unstable angina (UA), any repeat intervention inside the stent that had been implanted during the index procedure or within another segment or coronary vessel, stroke, and SVG surgery. Follow-up data were collected by telephone contact with the patient. No detailed workup for liver diseases was performed due to the emergency situation of AMI.
Dataset and statistics
The dataset (569 patients) included the socio-demographic parameters sex, age, and body mass index (BMI), as well as the serum parameters ALT, AST, bilirubin, CRP, creatinine, estimated glomerular filtration rate (eGFR), GGT, myoglobin, troponin, urea, potassium, and sodium.
Correlation analysis
Statistical data analyses were performed with R (http://www.r-project.org/). All data are presented as mean ± standard error of the mean (SEM) unless specified otherwise. Correlation analysis was performed using Spearman’s rank correlation coefficient r.
Importance analysis
For the importance analysis and building a predictive model, missing values were deleted. The remaining sample size was n = 406. The parameters were selected according to their importance obtained by an ensemble feature selection (EFS) approach. The EFS was calculated using the EFS-Server at http://efs.heiderlab.de. It provides an aggregation of eight feature selection methods, namely median, Pearson, and Spearman correlation, logistic regression, and four variable importance measures of the random forest algorithm [29]. We used the EFS server with default settings.
Predictive modeling
Predictive models were built up by a logistic regression as these models are easy to interpret and have been widely applied [30]. We used the implementation in the stats package of R (http://www.r-project.org) with standard settings. For evaluation of the classifier performance we followed the scheme of Sowa et al. [31]. A leave-one-out cross-validation scheme was used and the receiver operating characteristics (ROC) curve and the corresponding area under the curve (AUC) were calculated with pROC [32]. The 95% CI was computed with 2000 stratified bootstrap replicates. The ROC curve was built by plotting the sensitivity against the specificity for every possible cut-off between the two classes.
Results
Patient characteristics, basic parameters, and clinical outcome
Detailed data of the included patients grouped by survival can be found in Table I, comprising the distribution of socio-demographic parameters as well as serum parameters of heart, renal, and liver damage. Non-survivors were older and had significantly higher troponin, myoglobin, creatinine, urea, CRP, GGT, ALT, and AST levels. Non-survivors had significantly lower eGFR and prevalence of cardiovascular risk factors. Body mass index, bilirubin, and prevalence of STEMI or NSTEMI did not differ between the groups. Clinical follow-up was completed in 569 patients at 6 years (BMI: 27.5 ±0.2 kg/m2; age: 66.3 ±0.6; male/female: 406 (71.3%)/163 (28.7%)). Figure 2 depicts the unadjusted Kaplan-Meier curves of events up to 6 years after PCI. One hundred and fifty-six patients died during the follow-up period (Figure 2 A). During follow-up 65 cases of UA occurred (known incidence for 416 patients; Figure 2 B), 61 cases of restenosis (known for 416) were observed (Figure 2 C), and 32 reinfarctions were recorded (of 419; Figure 2 D). Stroke occurred in 12 (of 414 known) cases and an SVG surgery had to be performed in 17 (of 417) cases.
Table I
Demographic and basic parameters of the patient cohort at enrollment
Parameters | Non-survivors (total n = 156) | Survivors (total n = 413) | P-value |
---|---|---|---|
Age (n = 114 vs. 381) | 72.87 ±0.87 | 64.37 ±0.65 | < 0.01 |
Gender (n; female/male) | 53/103 | 110/303 | 0.096 |
BMI (n = 97 vs. 322) | 27.03 ±0.55 | 27.60 ±0.26 | 0.32 |
GGT (n = 156 vs. 411) | 70.54 ±9.0 | 46.20 ±2.50 | < 0.01 |
AST (n = 155 vs. 413) | 161.63 ±41.72 | 66.05 ±5.64 | < 0.01 |
ALT (n = 156 vs. 413) | 93.07 ±24.43 | 39.50 ±3.14 | < 0.01 |
Bilirubin (n = 152 vs. 385) | 0.65 ±0.03 | 0.65 ±0.02 | 0.95 |
CRP (n = 152 vs. 401) | 4.72 ±0.51 | 1.58 ±0.14 | < 0.01 |
Creatinine (n = 154 vs. 407) | 1.64 ±0.08 | 1.33 ±0.04 | < 0.01 |
Urea (n = 154 vs. 406) | 29.48 ±1.34 | 20.47 ±0.74 | < 0.01 |
eGFR (n = 113 vs. 378) | 49.60 ±1.87 | 61.41 ±0.91 | < 0.01 |
Troponin (n = 155 vs. 408) | 13.28 ±2.32 | 7.58 ±0.98 | < 0.01 |
Myoglobin (n = 146 vs. 381) | 639.29 ±132.78 | 301.60 ±31.97 | < 0.01 |
STEMI | 27 (26.7%)1 | 91 (27.5%)1 | 1F |
NSTEMI | 74 (73.3%)1 | 240 (72.5%)1 | |
Ex-/smoker (n = 100 vs. 324) | 12/15 (12%/15%) | 80/92 (24.7%/28.4%) | χ2: < 0.01 |
Type 2 diabetes | 28 (27.7% of n = 101) | 108 (33.4% of n = 323) | 0.3F |
Hypercholesterolemia | 73 (73% of n = 100) | 272 (83.6% of n = 325) | 0.0196F |
RR systolic (n = 97 vs. 324) | 127.4 | 134.6 | 0.01 |
RR diastolic (n = 97 vs. 324) | 63.4 | 66.3 | 0.1 |
Heart rate (n = 80 vs. 284) | 80.9 | 75.9 | 0.06 |
Family history | 18 (18.2% of n = 99) | 108 (33.5% of n = 322) | < 0.01F |
Continuous data are presented as mean ± SEM, categorical data as absolute numbers. Comparison between survivors and non-survivors by Student’s t test (continuous data) and Fisher’s exact test/χ2 (categorical data). ALT – alanine transaminase, AST – aspartate transaminase, BMI – body mass index, CRP – C-reactive protein, eGFR – estimated glomerular filtration rate, GGT – γ-glutamyl transferase.
Figure 2
Unadjusted survival curves and cumulative incidence of MACE. Incidence of cardiac death (A) is given over a follow-up time of 70 months. Only individuals with a known outcome are depicted. Deceased patients for whom time of death was not known were censored at 70 months. Cumulative incidences of major adverse cardiac events (MACE) are depicted for unstable angina (UA; B), restenosis (C), and reinfarction (D). Only individuals with known outcome were included in this analysis (351 for UA and reinfarction; 350 for restenosis). Patients for whom time of the MACE occurrence was not known were censored at 62 months (UA, restenosis) or 50 months (reinfarction)
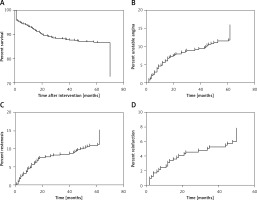
Age and non-invasive parameters correlate significantly with death after myocardial infarction
Outcome defined as death after AMI was correlated with demographic and serum-derived parameters. Detailed findings of this analysis are presented in Table II. Higher age significantly correlated with death. Also routine cardiac parameters (troponin, myoglobin) correlated significantly with death. Additionally, serum parameters usually not used in cardiac diagnosis (AST, creatinine, CRP, eGFR, sodium, and urea) correlated with death. Other non-invasive parameters did not exhibit significant correlations (Table II). Liver parameters (ALT, AST) can be elevated in consequence of reduced arterial perfusion or congestion due to acute cardiac failure. To assess whether this may be the case in the present cohort, in a subgroup of 90 patients, in whom transthoracic echocardiography (TTE) was available, signs of right ventricular load (RVL) were evaluated. In 12 patients with TTE a sign of RVL was found and 2 of these exhibited NAFLD. In the 78 patients without signs of RVL, 3 patients had alcoholic liver disease, 5 had an unidentified liver disease, and a high proportion exhibited signs of metabolic alterations (Table III). While ALT and AST were nominally higher in patients with RVL, significance was not reached compared to patients without RVL (Table IV).
Table II
Correlation of non-invasively determined parameters with death
Parameter | R1 | P-value |
---|---|---|
Age | –0.2874 | < 0.0001 |
ALT | –0.0199 | 0.6357 |
AST | 0.1377 | 0.001 |
Bilirubin | –0.0029 | 0.9470 |
Creatinine | 0.2384 | < 0.0001 |
CRP | 0.3114 | < 0.0001 |
eGFR | –0.268 | < 0.0001 |
Myoglobin | 0.2035 | < 0.0001 |
Sodium | –0.1313 | 0.0024 |
Troponin | 0.1167 | 0.0056 |
Urea | 0.3304 | < 0.0001 |
Table III
Clinical observations in 100 patients with available transthoracic echocardiography
Table IV
Serum liver injury parameters in patients with available transthoracic echocardiography grouped by signs of right heart burden
Parameter | Signs of right ventricular load present (n = 12) | No signs of right ventricular load present (n = 78) | P-value |
---|---|---|---|
AST [U/l] | 316.3 ±62.1 | 308.6 ±29.98 | 0.92 |
ALT [U/l] | 230.7 ±142.1 | 97.6 ±16.8 | 0.37 |
Correlations of serum parameters among each other
To assess whether non-invasive parameters for liver injury (ALT, AST, GGT) and routine heart parameters (troponin, myoglobin) were interrelated, correlation analysis was performed. All significant correlations are given in Table V. Both ALT and AST were correlated with myoglobin and troponin. In addition, GGT was inversely correlated with eGFR.
High importance of ALT and vascular parameters for prediction of death
To efficiently predict cardiac death after AMI an EFS approach was performed to select the most important non-invasive parameters for prediction. Detailed importance measures for all included parameters are given in Figure 3. A high importance (above the average importance = 0.41) could be identified for the variable age (0.76) as well as the serum parameters CRP (0.83), urea (0.73), eGFR (0.65), and myoglobin (0.49). The serum parameters ALT (0.4), creatinine (0.35), and sodium (0.37) reached reasonable importance (above [average – 1 × SEM] = 0.35), while none of the other parameters exhibited a high importance and thus were excluded from further analyses.
Figure 3
Ensemble feature selection importance analysis. The bars show the cumulative importance for the different parameters with respect to separation of death and survival. Higher numbers indicate greater importance for the classification. Partitions in the bars show contributions of single features of the ensemble selection approach to the total importance
P_cor – Pearson correlation, S_cor – Spearman correlation, LogReg – logistic regression, ER_RF – error rate of random forest, Gini_RF – Gini impurity in random forest, AUC_CF – area under the curve for conditional random forest, ER_CF – error rate of conditional random forest, eGFR – estimated glomerular filtration rate, Mygl – myoglobin, Trpn – troponin, Crtn – creatinine, CRP – C-reactive protein, Blrb – bilirubin, Gndr – gender.
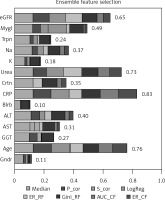
New diagnostic model for prediction of long-term death after acute myocardial infarction
Based only on parameters with an EFS importance above average (age, CRP, eGFR, myoglobin, and urea), a prediction model was developed based on a logistic regression model. It achieved an AUC of 77.6% (95% CI: 72.5–82.6%) (Figure 4 A). Separating deceased patients with known time of death (n = 76) by low and high CRP (cutoff at 4.2 mg/dl) resulted in significantly longer survival of patients with high CRP (Figure 4 B).
Figure 4
Performance of prediction model for prediction of cardiac death. (A) On the Y-axis the sensitivity and on the X-axis the specificity is shown. The ROC curve is shown as a solid line. The AUC of the prediction model is 77.6% (95% CI: 72.5–82.6%). The confidence interval is shown as a dashed line. The dotted line marks the performance of random guessing. (B) Kaplan-Meier analysis for C-reactive protein (CRP) as the most important parameter for prediction of death after acute myocardial infarction (AMI). Only patients with known time of death (n = 76) were included in the analysis and grouped according to low and high CRP (cutoff at 4.2 mg/dl). Patients with high CRP had significantly shorter survival time after AMI
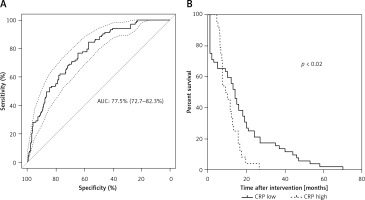
Discussion
In the present retrospective follow-up study, we have focused on patients after AMI and analyzed non-invasive markers for cardiac death. Using an EFS approach parameters with importance to predict long-term survival after AMI were identified and included not only classic cardiac markers, but also CRP, eGFR, and the classic liver parameter ALT. Additionally, liver parameters (ALT, AST and GGT) correlated significantly with heart parameters (myoglobin and troponin) and also with renal parameters (eGFR and urea). A prediction model was constructed with an AUC of 77.5% to predict cardiac death.
The parameters identified as important for non-invasive prediction of cardiac death corroborate previous findings of other groups. One of the most important factors identified was patient age, which is in line with the increased rate of mortality in elderly patients with AMI [33], and with a study of Dunn et al., comparing the survival of subjects with and without suspected NAFLD in a population-based cohort. Indeed, in this study, age was demonstrated to be a strong independent risk factor for cardiovascular death [34], similar to the cohort presented here. Age was the most important risk factor for in-hospital worsening of STEMI and distal embolization [35]. Also troponin was associated with cardiac death and relevant for prediction. In previous studies elevated troponin levels have been associated with a worse short- and long-term prognosis and the probability of death after AMI [36]. Another important factor for prediction of death after AMI was the eGFR. Again this is in line with previous findings, where renal impairment was associated with an increased risk of cardiac mortality [25, 37] and worse prognosis [38]. Serum creatinine and eGFR were identified as predictors of short- and long-term mortality in patients with AMI treated with primary angioplasty [38, 39]. Generally, patients with CKD tend to be older and have more cardiovascular risk factors and higher incidence of MACE [40].
Another finding of our study was that routine liver parameters were significantly correlated with classic cardiac markers and eGFR. This indicates the relevance of liver-derived parameters for cardiac outcomes. The extent of liver injury, in particular in settings with metabolic syndrome and/or NAFLD, may have a direct impact on cardiac outcomes. Previous studies demonstrated that elevation of transaminases is a common phenomenon in patients within the acute phase of AMI [41] and that elevated transaminases are associated with MACE [42]. The admission levels of transaminases were associated with complications and mortality during intensive care stay in a large study with patients mechanically reperfused for AMI [43]. In our study only ALT showed a high importance to predict mortality in patients after AMI in a long follow-up period. This is in line with a study of Yun et al. demonstrating that elevated ALT levels are independently associated with increased CVD-related mortality in Koreans [44]. Indeed, ALT is very specific for liver injury and also for NAFLD [45]. Unfortunately, in the present cohort no detailed data on liver-related morbidity, viral hepatitides or other liver diseases were available. Nevertheless, routine liver parameters might be able to improve prediction of cardiac outcome, though larger studies are needed to confirm this hypothesis.
One possible explanation for the importance of liver- and kidney-related serum parameters for survival in AMI might be common underlying risk factors: insulin resistance, hyperlipidaemia, abdominal obesity and hypertension. Metabolic syndrome basically comprises these risk factors for cardiovascular disease, NAFLD and subsequent CKD. There is also accumulating evidence that NAFLD is an early mediator of MS as a systemic disease [46], and NAFLD has been associated with cardiovascular disease and CKD in many studies [9, 47–49]. Furthermore, advanced fibrosis in NAFLD predicted not only liver-related mortality [50] but also increased mortality due to cardiovascular events [18, 51, 52]. Severity of NAFLD seems to be paralleled by the reduction of eGFR and severity of CKD [53, 54]. Although the exact underlying mechanism connecting elevated risk for CVD and CKD in NAFLD is still unknown, some data suggest a pivotal and causal role of NAFLD for more advanced metabolic injury, culminating in cardiovascular and renal outcomes [8, 20]. Apart from this, routine liver enzymes mostly exhibit no or only mild elevation in NAFLD [55, 56]. This could be one reason for the relatively low relevance of serum liver parameters for prediction of cardiac mortality in this study. One limitation of the present study is that no detailed information on metabolic status or liver steatosis/NAFLD was present, as these are not routinely diagnosed in the emergency setting of AMI. This could be one explanation for the lower incidence of components of the metabolic syndrome in non-surviving patients. The proportion of individuals for whom this information was available differed between the groups. Another reason for this observation could be a more rigorous follow-up and faster response to any adverse events in patients with metabolic disturbances, leading to better survival. However, this is speculative and would need confirmation in prospective studies.
To predict mortality after AMI other scoring systems are in use, which mostly rely on parameters available during percutaneous intervention. These scores reach AUCs of up to 85% for prediction of 1-year mortality [39, 57]. In our study a scoring system comprising only non-invasive parameters reached an AUC of 77.6% for prediction of 6-year mortality. The best performance for commonly used scores for 6-year mortality (GRACE score) was also 77%. It is interesting to note that diagnostic criteria of the metabolic syndrome and body fat distribution can predict the severity of an AMI, but not the outcome after the AMI [58]. One limitation of our study is that the time point of death was not known for all included patients. Another limitation is that the cause of death was not known in the majority of cases. Thus, the presented score should be interpreted as a prediction of overall mortality, independent of survival time (no separation of short- or long-term mortality or by cause of death). Due to the non-invasive nature of all used parameters, monitoring of cardiac health could be performed based on this or similar systems.
In conclusion, besides age, heart and renal parameters, liver parameters were associated with cardiac death and identified as possibly important for discrimination of non-survivors and survivors in a long-term follow-up of patients after AMI. On the one hand, cardiometabolic risk factors and common pathogenic mechanisms for CVD, CKD, and NAFLD should be analyzed for predictive properties and disease monitoring in this setting. On the other hand, a causal role for (an unknown) underlying liver disease should be considered to identify early markers of long-term cardiac outcomes.