Purpose
Brachytherapy (BT) refers to a specific form of radiotherapy consisting of a precise placement of radiation source directly into or next to the tumor, to safely deliver sufficient radiation doses for tumor eradication [1]. Brachytherapy, as an addition to external beam radiation therapy (EBRT), is mainly indicated for: 1) Patients with locally advanced cervical and vaginal cancers to be used in combination with chemotherapy; 2) Patients with high-risk prostate cancer to escalate radiation dose and improve progression-free survival; 3) Surgically treated patients with endometrial cancer to decrease the risk of vaginal recurrence; and 4) Medically inoperable colorectal cancer patients [2-5]. Brachytherapy is also an effective complementary radiotherapeutic modality for other cancer sites, including breast, brain, head and neck, bronchus, and esophagus [6]. Brachytherapy can be delivered either as low-dose-rate (LDR), high-dose-rate (HDR), or medium-dose-rate (MDR) therapies. Pulsed-dose-rate (PDR) is an alternative form of BT, in which the radiation is carried out over a more extended period by delivering radiation dose in several intermittent small radiation fractions [7]. Brachytherapy with or without supplemental EBRT has demonstrated excellent tumor control [6]. However, quality of life after BT treatments has become a concerning issue among patients and physicians due to potential toxic effects of BT on surrounding normal tissues.
Since pelvic tumors are close to the rectum, rectal toxicity remains a serious side effect in patients treated with radiation therapy. Rectal toxicity manifests in different grades, ranging from mild proctitis to more severe cases of ulceration, bleeding, fistula formation, and death [8]. Due to the lack of active diagnosis, rectal toxicity may be under-recognized and detected at advanced grades when the complications are very detrimental in daily activities of the patients. Although the incidence of grade ≥ 2 rectal toxicity following BT is typically within the range of 5-7% [8], this risk may be increased when BT is combined with EBRT [9]. Since supportive medical management is the only treatment option for rectal injuries (e.g., laxatives, hydration, argon plasma coagulation, or surgery) [10, 11], it would be beneficial to identify high-risk patients, who could benefit from preventive modalities (e.g., rectal spacer placement) [12].
Clinical prediction models are mathematical tools designed to discover the relationship between baseline clinical status (starting point) and future outcomes (endpoints) [13]. They can estimate objective individualized risk of developing treatment side effects, while avoiding common biases observed in clinical decision making [14]. Conversely, prediction models are susceptible to biases related to data collection, modeling methodology, performance measurement, and model presentation [15]. Since data generation in healthcare is outstripping the capacity of human cognition to adequately manage all these data, machine learning can provide a scalable way to manage the growing data and decision complexities.
The growing number of recent publications for predicting the risk of rectal toxicity highlights clinical demand to identify patients who are at greatest risk of developing radiation-induced rectal side effects. However, to date, there has not been a formal synthesis or quality assessment of existing prediction models, which is essential to determine whether they could be used for decision-making and guide development of future models. This study aimed to: 1) Identify the available prediction models for rectal toxicity in patients who received brachytherapy in the abdominal pelvic area; 2) Identify the candidate and significant risk factors for rectal toxicity; and 3) Evaluate the risk of bias and applicability of prediction models to discuss possible future directions.
Material and methods
Systematic literature search
A systematic literature search was performed using MEDLINE (via PubMed) database. To increase the clinical relevance of the findings, we only included papers published from January 1, 1995 up to August 31, 2021. The medical subject heading (MeSH) terms for “pelvic cancers”, “brachytherapy”, “prediction models”, and “rectal toxicity” were combined using logic operators (see Supplementary Material S1 for the detailed search strategy). Further to using the above search database, reference lists of included studies and relevant reviews were also explored for additional publications. This study was performed in accordance with preferred reporting items for systematic reviews and meta-analyses (PRISMA) [16].
Eligibility criteria and study selection
The aim of our search was to identify studies that developed prediction models, which provided personalized estimates of rectal toxicity after brachytherapy in patients with any types of pelvic cancers (i.e., prostate, cervix, vagina, endometrium, bladder, rectum, or anus). External validation studies were also eligible for inclusion. Only papers written in the English language were included. The following criteria were used to exclude irrelevant studies: only a subset of patients who received BT, no multivariate analysis due to small number of events, lack of model specification, case mix studies, no significant predictors in multivariate analysis, or univariate-only analysis.
The screening process consisted of two phases. Preliminary screening was carried out through reviewing the titles and abstracts by two independent reviewers (FT and YW) with backgrounds in oncology and machine learning. In the second phase, the reviewers independently screened full texts of the selected studies using the predefined eligibility criteria. Discrepancies between reviewers were resolved by consensus.
Data extraction
A data extraction form was developed to collect all relevant information based on recommendations in CHARMS checklist (see Supplementary Material S2 for the data extraction form) [17]. The following key items were extracted from the included studies: publication year, country, source of data, age, sample size, cancer site, type of BT (LDR, HDR, or PDR), EBRT (yes or no), chemotherapy (yes or no), outcome, measuring standard of the outcome, time of outcome assessment, number of events, candidate predictors, effect estimate of significant risk factors, modeling technique, performance measures, and study limitations.
Quality assessment
Prediction model risk of bias assessment tool (PROBAST) was used to assess risk of bias (ROB) of each prediction model [18]. PROBAST is based on 20 signaling questions grouped into four domains, including participants, predictors, outcome, and analysis. Each signaling question is judged as “yes”, “no”, “probably yes”, “probably no”, and “no information”. The questions facilitate reaching the overall judgement of risk of bias for each model (low-risk, high-risk, or unclear). Applicability was also assessed as being of low, high or unclear concern.
Results
As shown in Figure 1, 6,018 studies were identified through systematic and manual searches, of which 129 studies were eligible for inclusion after title and abstract screening. During the full-text screening, 99 papers failed to meet the minimum requirements for review and were excluded, resulting in 30 articles. There was no independent external validation study for the included models.
Characteristics of the included studies
As shown in Table 1 [19-48], 16 (54%) studies described prediction models for patients with cervical cancer [19-34], and 13 (44%) studies included the prostate cancer patients [35-47]. Moreover, one study developed a prediction model for elderly inoperable rectal cancer patients [48]. The studies were published between 1999 and 2019, with a median sample size of 221 (IQR: 96-617) for cervical cancer and 503 (IQR: 165-2,088) for prostate cancer. The studies were carried out mostly in the United States (n = 7, 23%) [19, 35-37, 40, 41, 47], followed by Taiwan (n = 6, 20%) [21-23, 26, 27, 29], Japan (n = 5, 17%) [25, 38, 42, 44, 46], and South Korea (n = 4, 13%) [28, 30, 31, 43].
Table 1
Characteristics of the studies for predicting brachytherapy-induced rectal toxicity in patients with pelvic cancers
Study | Year | Country | Sample size | BT type | Outcome | Incidence of RT (%) | Acute/late toxicity | Modeling technique | Model evaluation | Limitation(s) | |
---|---|---|---|---|---|---|---|---|---|---|---|
Cervix | Perez et al. [19] | 1999 | USA | 1,456 | LDR | Rectosigmoid toxicity | 7.0 | Late | Cox | – | 1. No detailed toxicity assessment, 2. Lack of acute events |
Barillot et al. [20] | 2000 | France | 642 | HDR | Rectal toxicity | 21.5 | Late | Logistic | – | N.R. | |
Chen et al. [21] | 2000 | Taiwan | 128 | HDR | Rectal toxicity | 29.7 | Late | Logistic | – | 1. Not addressing the irradiated volume of the rectum | |
Chen et al. [22] | 2004 | Taiwan | 154 | HDR | Rectal toxicity | 24.7 | Late | Logistic | – | 1. Not addressing the irradiated volume of the rectum | |
Wang et al. [23] | 2004 | Taiwan | 541 | HDR | Proctitis | 37.2 | Late | Cox | – | 1. Results limited to low grade toxicities | |
Saibishkumar et al. [24] | 2006 | India | 1,069 | HDR/ LDR | Rectal toxicity | 12.3 | Late | Logistic | – | 1. Retrospective design | |
Noda et al. [25] | 2007 | Japan | 92 | HDR | Rectal toxicity | 26.1 | Late | Logistic | – | 1. CT was obtained only in the first session of brachytherapy, 2. Outer surface of the rectal wall was considered as reference point, 3. Lack of rectal volume measures | |
Chen et al. [26] | 2009 | Taiwan | 392 | HDR | Rectal toxicity | 11.7 | Late | Cox | – | 1. Not addressing the irradiated volume of the rectum | |
Chen et al. [27] | 2010 | Taiwan | 212 | HDR | Rectal toxicity | 19.8 | Late | Logistic | – | N.R. | |
Kang et al. [28] | 2010 | South Korea | 230 | HDR | Rectal bleeding | 43.0 | Late | Cox | – | 1. Different outcome assessments, 2. Changes in EBRT over time, 3. Lack of dose optimization for all patients | |
Huang et al. [29] | 2013 | Taiwan | 267 | HDR | Proctitis | 12.0 | Late | Cox | – | 1. One dosimetric planning at the beginning of BT, 2. Few grade 3-4 toxicity events | |
Kim et al. [30] | 2013 | South Korea | 77 | HDR | Rectal toxicity | 28.6 | Late | Logistic | – | 1. 36.4% of patients completed only 2-3 out of 4 examinations | |
Kim et al. [31] | 2015 | South Korea | 1,559 | HDR | Rectal toxicity | 8.9 | Late | Cox | – | 1. Retrospective design, 2. Underestimation of events | |
Ujaimi et al. [32] | 2017 | Canada | 106 | PDR | Rectal toxicity | 34.9 | Late | Logistic | AUC: 0.77 | 1. Retrospective design, 2. Moderate sample size, 3. OARs’ movement during PDR-BT, 4. Lack of patient-reported outcomes | |
Zhen et al. [33] | 2017 | China | 42 | HDR | Rectal toxicity | 28.6 | Late | CNN | AUC: 0.58 | 1. Limited sample size | |
Chen et al. [34] | 2018 | China | 42 | HDR | Rectal toxicity | 28.6 | N.A. | SVM | AUC: 0.91 | 1. Small sample size, 2. Large number of input features, 3. Lack of clinical variables | |
Prostate | Merrick et al. [35] | 2003 | USA | 213 | LDR | Rectal toxicity | N.A. | Late | Linear | – | N.R. |
Bittner et al. [36] | 2008 | USA | 548 | LDR | Rectal bleeding | 6.6 | Late | Logistic | – | 1. Different EBRT and BT doses | |
Zelefsky et al. [37] | 2008 | USA | 127 | LDR | Rectal toxicity | 11.8 | Late | Logistic | – | 1. Low incidence of acute toxicities | |
Shiraishi et al. [38] | 2011 | Japan | 458 | LDR | Rectal toxicity | 9.6 | Late | Logistic | – | 1. Retrospective design, 2. No 103Pd was used, 3. No EBRT, 4. Many patients excluded from analysis | |
Keyes et al. [39] | 2012 | Canada | 1,006 | LDR | Rectal toxicity | 8.0 | Acute | Cox | – | 1. Lack of precise dose calculation after BT, 2. No patient-reported outcomes | |
Buckstein et al. [40] | 2013 | USA | 2,046 | LDR | Rectal toxicity | 4.5 | Late | Cox | – | 1. Retrospective design, 2. Less commonly outcome scales, 3. Underestimation of events | |
Price et al. [41] | 2013 | USA | 2,752 | LDR | Proctitis | 6.4 | Late | Cox | – | 1. Retrospective design, 2. Underestimation of events | |
Shiraishi et al. [42] | 2013 | Japan | 369 | LDR | Rectal bleeding | 10.3 | Late | Logistic | – | 1. Uncertainty associated with the estimate of/for late rectal toxicity | |
Kang et al. [43] | 2015 | South Korea | 178 | LDR | Rectal toxicity | 12.9 | Late | Logistic | – | 1. Moderate sample size, 2. Short follow-up period | |
Katayama et al. [44] | 2016 | Japan | 2,339 | LDR | Rectal toxicity | 2.9 | Late | Cox | – | 1. Inter-observer variability in post-implant dosimetry, 2. Lack of EBRT DVH parameters, 3. Short follow-up period | |
Kragelj et al. [45] | 2017 | Slovenia | 77 | HDR | Rectal toxicity | 39.0 | Late | Logistic | AUC: 0.7 | 1. Inconsistency of the study instrument, 2. Missing patient-reported outcomes, 3. > 50% baseline defecation problem | |
Tanaka et al. [46] | 2018 | Japan | 2,216 | LDR | Rectal toxicity | 5.7 | Late | Cox | – | 1. Single-institution and retrospective design, 2. Different outcome scales, 3. Lack of repeated measured outcomes | |
Ling et al. [47] | 2019 | USA | 620 | LDR | Rectal bleeding | 12.4 | Late | Cox | – | 1. Imperfect response rate for the EPIC questionnaire | |
Rectum | Rijkmans et al. [48] | 2019 | The Netherlands | 25 | HDR | Proctitis | 40.0 | Late | Cox | – | 1. Small sample size, 2. Confounding effect of residual tumor and tumor regression |
[i] AUC – area under the receiver operating characteristic curve; BT – brachytherapy; CT – computed tomography; CNN – convolutional neural network; EBRT – external beam radiation therapy; EPIC – expanded prostate cancer index composite; HDR – high-dose-rate; LDR – low-dose-rate; N.A. – not applicable; N.R. – not reported; OAR – organ at risk; PDR – pulsed-dose-rate; RT – rectal toxicity; SVM – support vector machine; USA – United States of America
Figure 2 shows a summary of the included prediction models. In terms of BT technique, 15 studies performed HDR-BT (n = 13 cervix, n = 1 prostate, and n = 1 rectal cancer) [20-23, 25-31, 33, 34, 45, 48], 13 studies used LDR-BT (n = 12 prostate and n = 1 cervical cancer) [19, 35-44, 46, 47], one study applied PDR-BT for cervical cancer patients [32], and one study included cervical cancer patients treated with either HDR-BT or LDR-BT [24]. Two studies (7%) excluded patients who received EBRT [39, 43], and 11 studies (37%) included patients who were treated with chemotherapy (n = 8 concurrent, n = 2 adjuvant, and n = 1 neoadjuvant) [19, 21-23, 26-32].
Fig. 2
Overview of the identified prediction models for brachytherapy-induced rectal toxicity in patients with pelvic cancers
HDR – high-dose-rate; LDR – low-dose-rate; PDR – pulsed-dose-rate
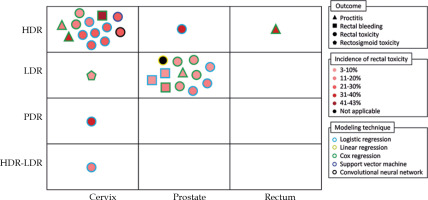
The majority of the studies (n = 28, 93%) used regression as machine learning algorithm (n = 14 logistic, n = 13 Cox, and n = 1 linear). One study applied support vector machine (SVM) to develop a rectal dose-toxicity model based on both dose map features and dose-volume histogram [34]. Moreover, one study applied convolutional neural network (CNN) to predict the probability of rectal toxicity based on dose distribution of the planning images [33]. Only four studies (13%) internally evaluated the predictive power of prediction models in terms of area under curve (AUC) for receiver operating characteristic (ROC), ranging from 0.58 to 0.91 [32-34, 45].
Candidate and significant predictors
Models were developed using 60 distinct predictors. As shown in Figure 3, following variables were the most common candidate predictors: age (n = 14, 47%), tumor stage (n = 10, 33%), EBRT (n = 6, 20%), V100% rectum (BT) (n = 6, 20%), and diabetes (type 1 or 2) (n = 5, 17%). Moreover, androgen deprivation therapy was considered in five (38%) prediction models for prostate cancer patients. The most common predictors retained in the final models were age (n = 5, 17%), EBRT (n = 5, 17%), V100% rectum (BT) (n = 5, 17%), and dose at rectal point (n = 3, 10%).
Outcome assessment
The following outcomes were considered as the primary endpoint of prediction models: proctitis (n = 4, 13%) [23, 29, 41, 48], rectal bleeding (n = 4, 13%) [28, 36, 42, 47], and recto-sigmoid toxicity (n = 1, 3%) [19]. Furthermore, 21 (70%) studies measured all types of rectal toxicity events [20-22, 24-27, 30-35, 37-40, 43-46]. Radiation Therapy Oncology Group (RTOG) scale (n = 19, 63%) [19, 20, 22-30, 36, 38-43, 45] and common terminology criteria for adverse events (CTCAE) (n = 7, 23%) [31, 32, 34, 37, 44, 46, 47] were the most common outcome measuring standards. Only one study evaluated acute rectal toxicity events during the first 6 weeks after BT [39]. The median incidence of rectal toxicity in studies, which included cervical and prostate cancer patients was 25.4% (IQR: 12.1-29.4%) and 8.8% (IQR: 5.9-12.3%), respectively.
Methodological limitations
Authors declared the following limitations, which might affect the validity and generalizability of their models: dose calculation uncertainties (n = 7, 23%) [25, 28, 29, 32, 39, 42, 44], retrospective design (n = 7, 23%) [24, 31, 32, 38, 40, 41, 46], limited sample size (n = 5, 17%) [32-34, 43, 48], lack of specific potential predictors (n = 3, 10%) [25, 34, 44], underestimation of toxicity events (n = 3, 10%) [31, 40, 41], lack of addressing irradiated volume of the rectum (n = 3, 10%) [21, 22, 26], and low incidence of grade 3-4 toxicity events (n = 2, 7%) [23, 29]. Moreover, 11 (37%) studies pointed out the outcome assessment challenges (e.g., short follow-up, lack of patient-reported outcomes, or change of measuring standards over time) [19, 28, 30, 32, 39, 40, 43-47].
Quality assessment
Summary of ROB and applicability of prediction models are shown in Table 2. Twenty-one (70%), three (10%), and one (3%) models were at low ROB for participants, predictors, and outcome, respectively, but none of the models were considered to be at low ROB for analysis. Common source of population bias was inappropriate or lack of information on inclusion/exclusion criteria (n = 8, 27%) [20, 31, 33, 34, 37, 41, 45, 46]. The main concerning issue with regards to the predictors domain was lack of information about knowledge of outcome during predictor assessment (n = 20, 67%) [20-28, 31-35, 37, 39-41, 44, 46]. Within the outcomes domain, sources of bias included subjective outcome assessment [23, 28, 31, 38, 42, 43, 45, 47], and lack of information on whether the outcome assessor was informed about the predictors or not [19, 21-30, 32-35, 37, 39-41, 44, 46].
Table 2
Risk of bias and applicability concern of the prediction models for brachytherapy-induced rectal toxicity in patients with pelvic cancers
[i] ROB – risk of bias; N.A. – not applicable; * non-regression modeling technique was used, for which PROBAST could not be applied on all domains; + indicates low ROB/low concern regarding applicability; – indicates high ROB/high concern regarding applicability; ? indicates unclear ROB/unclear concern regarding applicability
ROB in the analysis domain was the major contributor to the overall high ROB. Five (17%) models were likely overfitted due to a low event per candidate predictor ratio [29, 30, 34, 39, 48]. Twelve (40%) studies did not handle the continuous variables appropriately (i.e., dichotomized into ≥ 2 categories) [21, 22, 25-30, 32, 37, 40, 41]. None of the studies provided explicit mention of the methods used to handle missing data. More than half of the studies (n = 19, 63%) performed univariable predictor selection [20, 21, 23, 25, 28, 30-32, 36, 38-42, 44-48]. Only seven (23%) studies used survival analysis appropriately accounting for censoring [22-24, 28, 37, 40, 44]. Performance measure was only reported in four studies, which was limited to AUC, and none of them considered optimism-correction or penalization of parameters [32-34, 45]. Twenty-five studies (83%) appropriately presented regression coefficients corresponding to reported results from multivariable analysis [19-22, 24-28, 30-32, 35-47]. It should be noted that ROB of the analysis domain for two studies, which used non-regression modeling techniques was scored as “not applicable” [33, 34]. In terms of applicability, 22 (73%), 20 (67%), and 18 (60%) studies were applicable to the review question in participants, predictors, and outcome domains, respectively.
Discussion
Interpretation of the findings
We identified 30 prediction models featuring 60 distinct predictors for rectal toxicity after brachytherapy in patients with pelvic cancers (n = 16 cervix, n = 13 prostate, and n = 1 rectal cancer). The following variables were more markedly associated with rectal toxicity: age, EBRT, V100% rectum (BT), dose at rectal point, tumor stage, baseline bladder complications, biologically effective dose (EBRT + BT) using 3 for α/β, and mean dose to the parametrium. Although an enormous effort has been made to identify risk factors for brachytherapy-induced rectal toxicity, caution should be used when considering the application of these models in clinical practice.
In the field of machine learning, the use of different outcome events (e.g. proctitis, bleeding, etc.), measuring standards (e.g., RTOG, CTCAE, etc.), and timepoints (acute and late) negatively affected the re-usability and comparability of prediction models. This review shows the paucity of a comprehensive instrument for assessing radiation-induced rectal toxicity events. Developing a comprehensive scoring system, including relevant anatomical sites (i.e., rectum, sigmoid, and anus), accompanied by an administration protocol with instructions for outcome assessment and analysis, would be of great importance in improving the quality of future prediction models.
Due to the relatively low incidence of rectal toxicity events, overfitting remains as the most concerning risk in model development studies. In datasets with few events, standard regression methods could accurately predict outcomes for patients in training dataset, but often perform less accurately in a new group of patients. This difference is because the fitted model captures not only the underlying clinical associations between the predictors and outcome, but also the random variations in data. Using penalized regression (i.e., least absolute shrinkage and selection operator, ridge and elastic net regression) is one solution to deal with few number of events [49]. However, it is still recommended that the number of events relative to the number of candidate predictors should be greater than or equal to 20 [50]. Furthermore, active measurements of patient-reported outcomes could also address the underestimation of rectal toxicity events.
Predictors included in the models should be accurately measured and reliable. Uncertainties in dose calculation and lack of potential covariates generally dilute the predictive power of the model. A prospective study with a well-planned data collection protocol is an ideal solution to minimize measurements’ bias. However, this approach is not possible in all circumstances due to time and resource limitations. Retrospective design is a more convenient and relatively inexpensive strategy to utilize the readily existing data, and easily collect the conditions where there is a long latency between exposure and disease and to perform studies of rare events. Notwithstanding the advantages, the following issues should be considered when performing retrospective data collection: unrecoverable or unrecorded data items, difficult interpretation of information in data (e.g., acronyms, jargon, photocopies, and micro-fiches), difficult exploration of causes and effects, problematic verification of information, variance in the quality of information recorded by different medical professionals, and historical threat of changes in interventions and exposures [51]. In addition, predictors should be easy to measure and readily available in routine practice. Moderately predictive covariates that require additional time and measurement efforts, would not be easy to apply for screening, and thus not cost-effective [52].
Predictive performance is a multi-faceted concept that should be presented in terms of detailed discrimination, calibration, and overall performance indices. C-statistic or AUC itself is insensitive, which means it hardly changes even when very strong predictors are added/removed in/from the model [53]. The re-classification table, net re-classification improvement, and integrated discrimination improvement, which are refinements of discrimination and claim to move beyond the AUC, have therefore been proposed [54]. Calibration is another important criterion that refers to the agreement between individual risk predictions and observed outcomes. Models must be well-calibrated to support decision-making at patient level. Calibration drifts easily over time and across different clinical settings. Therefore, it is necessary to not only measure calibration on the development data, but also re-calibrate the models regularly before clinical use [55, 56]. Decision-curve analysis is also a relatively novel method to quantify the clinical usefulness of a prediction model. Interpretation of the decision curve is based on comparing the net benefit of a model with that of a strategy of “treat all” and “treat none”, where net benefit is a function of relative harms of false negatives and false positives [57].
Although more than half of the studies were considered to have low concern for applicability to our review question in participants, predictors and outcome domains, ROB, was not satisfactory due to issues related to the analysis domain. The following three deficiencies were the main reasons for the overall high ROB judgment.
First, inappropriate handling of continuous variables. The usual fallacious reason is that the dichotomization (categorization) of continuous variables maintains simplicity, and facilitates clinical interpretation. However, it leads to loss of information and substantially reduced predictive ability [58].
Second, using univariate analysis as the predictor selection technique. This method can result in incorrect predictor selection because variables are chosen according to their effect as a single predictor, rather than in context with other predictors. Analysis bias occurs because some predictors show their predictive value only after adjustment for other predictors [59]. Previously known important predictors may not reach statistical significance due to data shortfalls (e.g., small sample size). In addition, non-predictive variables may be selected based on a spurious association in the development dataset. A better approach is to make decisions on removing, including, or combining candidate predictors based on non-statistical methods (i.e., existing knowledge in the literature in combination with applicability, availability, reliability, and measuring cost relevant to the targeted setting) [60]. Alternatively, statistical methods that are not based on prior statistical tests can be used to reduce the dimensionality of data, such as principal components analysis.
Third, ignoring complexities and assumptions. Here, we indicate some key considerations related to study design and analysis complexities: (1) If a case-control design is used as the development dataset, control participants must be weighted by the inverse of their sampling fraction, otherwise the predicted probability would be biased [61]; 2) Since rectal toxicity symptoms usually manifest months after BT, appropriate time-to-event analysis (e.g., Cox regression) should be applied to correctly deal with the censored participants. The use of a logistic regression model that simply excludes censored participants leads to an unbalanced dataset that includes fewer persons without the outcome [62]; 3) Since each patient can experience more than one event of rectal toxicity, correct modeling methods, including multilevel or random-effect logistic or Cox regression, are needed to avoid bias in effects of predictors [63].
Study limitations
The following limitations should be declared. First, search was limited to the papers written in the English language without considering the gray literature. However, the missing models due to this are usually of relatively low quality and limited in usage. Second, a quantitative synthesis of predictors or AUCs was not performed due to the heterogeneity of predictors and participants.
Implications for future research
Since the principal aim of prediction models for calculating the risk of rectal toxicity is clinical integration, collaborative clinical and technical efforts are needed to make the models reliable, transparent, and easy-to-use in daily practice. As of today, a great amount of machine learning-based concepts and instruments serve as a standard for data cleaning, augmenting, transforming as well as exploring linear and non-linear associations. However, barriers to robust implementation of machine learning products still remain. The following three steps provide the path to achieve the primary goal of providing useful individually-tailored predictions via point-of-care decision support systems: 1) Evaluation of the model impact in the form of a randomized controlled trial, as the highest level in the of hierarchy of evidence, would increase clinician confidence to use prediction tools in the clinic [64]; 2) Using a unique language for communicating the models’ specifications and performance would foster the appraisal and synthesis of the prediction models [65]; and 3) Continuous learning is of paramount importance, in which the models dynamically learn and evolve their behavior based on new input data while retaining previously-learned associations [66].
Conclusions
The findings of this review indicate several methodological drawbacks. The studies reviewed here should be understood as an initial attempt to begin a more systematic approach for developing more robust prediction models in the future. We suggest future investigators to measure patient-reported outcomes to address underestimation of the rectal toxicity events, provide higher priority to reliable dose-volume parameters, avoid overfitting by considering an event per candidate predictor rate ≥ 20, and calculate detailed performance measures in terms of discrimination, calibration, and decision analysis. Further efforts are needed to boost the application of prediction models in selecting patients who are at high-risk of developing brachytherapy-induced rectal toxicity, and can benefit from preventive or alternative cancer treatments.