INTRODUCTION
Traumatic brain injury (TBI) has been recognized as a “signature wound” of the military during the wars of the 21st century [1]. Somatic and mental consequences of TBI may persist even years after a traumatic event, significantly reducing quality of life and social functioning [2]. It remains an influential factor for veterans’ mental health, increasing the prevalence of posttraumatic stress disorder (PTSD), anxiety and depressive disorders, substance use disorders and the number of suicide attempts [3]. Therefore, it is important to study the processes related to the reduction of TBI symptoms [2].
Psychological processes are considered to be important for symptom reduction or chronicity – years after a traumatic event TBI symptoms are still highly affected by ineffective adjustment mechanisms that provoke chronic stress conditions, which, in turn, make symptoms of TBI persistent [4]. An important concept linked to psychological adaptation in TBI is resilience. This is a process that reflects the dynamic ability of a person to restore adaptive and effective psychosocial functioning and grow personally, following a period of maladaptation occurring due to the disorganizing effect of traumatic factors [5]. A meta-analysis conducted by Färber et al. shows that resilience is associated with better mental health outcomes in patients with somatic diseases [6]. It was found that the resilience of veterans with TBI is lower than in those without head trauma; however, the mechanisms and factors underlying this are yet to be discovered [7]. These facts become significant in the light of findings that suggest resilience may promote recovery in veterans with TBI [8, 9].
In recent years new theories of resilience have been developed that could encourage further studies. They see resilience as more dependent on subjective and psychological factors than on hard-to-change personality traits or socio-demographic factors. For example, Nalder et al. presented a theoretical resilience model in patients with TBI, which describes resilience as a complex process in which TBI-related adversities form an initial response which then is shaped by cognitive, emotional, and behavioral factors and self-regulatory processes to manage the adversities faced and reach resilience-related outcomes [10]. Stainton et al. also proposed a processual theoretical model suggesting that resilience is a dynamic process of utilizing different protective factors (of social, neurobiological, cognitive, and psychological nature) that are of benefit to the patient’s mental health [11]. What we found interesting in these models is that they pay much more attention to cognitive and emotional factors as potentially more valuable for resilience than to clinical indicators or demographic characteristics. However, these authors also argue that their models lack empirical evidence, and future empirical research should identify different protective factors and the ways they interact and predict resilience [10, 11].
In our recent review we analyzed the state of knowledge on resilience factors in veterans with persistent TBI symptoms [12]. While sociodemographic and personality factors of resilience were previously studied in veterans with TBI, a small number of studies described the relationship between resilience and different clinical variables like persistent TBI symptoms or post-traumatic stress symptoms, and we found no studies describing the relationship between resilience, cognition, and emotions in veterans years after head trauma, despite their importance in new theoretical models of resilience in TBI [12]. We also found no studies describing the value of different resilience predictors together [12].
The concept of resilience is therefore still unstable and not well defined, so it is of great scientific interest to study the processes underlying resilience and important variables in veterans with persistent brain injury symptoms [7, 11, 13]. Resilience is a multi-dimensional construct and to obtain its full-scale characteristics it is important to assess the relationship of resilience to different variables to distinguish those that are most valuable [14-16]. These circumstances indicate the need for research aimed at studying resilience characteristics in veterans with persistent traumatic brain injury symptoms, its relationship with the course and severity of TBI, and socio-demographic, clinical, cognitive, and affective symptoms.
The aim of the study was to understand the relationships between resilience and socio-demographic, clinical, cognitive, and affective variables in veterans with traumatic brain injury in the remote period and to identify and estimate the value of different resilience predictors.
We hypothesized that:
resilience in veterans with remote TBI has a stronger correlation with clinical, cognitive and affective variables than with socio-demographic factors and trauma characteristics;
a set of cognitive and affective variables may explain most of the variability in resilience even after exclusion of other variables.
METHODS
Participants and procedure
The cross-sectional study of Ukrainian veterans of an anti-terrorist/Joint Forces Operation with persistent TBI symptoms (disturbing the patient more than 3 years after the event concerned) was conducted in Ukraine from 2019 to 2021. The local committee of bioethical expertise and ethics of scientific research approved all procedures of the study (protocol №127 from 02.12.2019), which was conducted as part of the research program “Dynamic biopsychosocial model of medical and psychological care (diagnosis, therapy, rehabilitation, prevention) of patients of multidisciplinary hospitals in a rapidly changing crisis-associated society” (registration №0119U103910).
Participants were recruited in Kyiv veterans’ healthcare system facilities. Written informed consent was obtained from every participant at the beginning of the assessment. The objectives of the study and procedure were clearly explained prior the assessment. All materials were administered in paper form. Only the principal researcher had access to the personal information of the participants in the study.
The diagnosis of mild TBI (concussion and mild cerebral contusion) in all patients was established earlier during the acute period of trauma in military hospitals via clinical and instrumental examination, with documentary evidence. Assessment of the patient’s neurological condition and confirmation of the persistence of symptoms and diagnosis of previous TBI at the time of involvement in the study was performed by a neurologist via examination and review of medical records of an earlier period of injury. All trauma types were of mild severity according to the VA/DOD Clinical practice guideline for the management of mild TBI (immediately after trauma GCS was 13-15, loss of consciousness time up to 30 minutes, alteration of consciousness time up to 24 hours, post-traumatic amnesia period up to 1 day [17]).
The assessment included collecting information about socio-demographic characteristics, psychological assessment for resilience, neurobehavioral symptoms, post-traumatic stress, cognitive performance, anxiety, depression, positive and negative affect, and quality of life. It took from 30 to 60 minutes to be completed by each participant.
Measures
To broadly assess socio-demographic, clinical, cognitive and emotional variables, we used a battery of well-known questionnaires, translated into Ukrainian. Resilience was measured with Connor-Davidson Resilience Scale (CD-RISC). To assess the most common clinical issues in veterans with TBI the Neurobehavioral symptom inventory (NSI), post-traumatic stress disorder checklist 5 edition (PCL-5), the hospital anxiety and depressive scale (HADS) and Chaban quality of life scale (CQLS) were used. The Montreal cognitive assessment scale (MoCA) was used to evaluate cognition. To assess affective variables, we used the positive and negative affect scale (PANAS). Detailed descriptions of the instruments used:
Connor-Davidson Resilience Scale (CD-RISC) developed by K.M. Connor and J.R.T. Davidson, the scale contains 25 statements, each to be evaluated on a five-point Likert scale from 0 to 4. The minimum possible score is 0, the maximum possible score is 100 points, with a higher score representing better resilience. The CD-RISC scale is a valid, internally consistent (α = 0.89) and reliable measure of resilience [18]. Translation and adaptation into Ukrainian were performed by K. Kolesnyk and Y. Batrakova [19].
Neurobehavioral Symptom Inventory (NSI) is an inventory, developed by K.D. Cicerone and K. Kalmar. The NSI is a self-questionnaire containing 22 statements about somatic, affective, sensory, and cognitive clusters of post-concussion syndrome [20], with total score ranges from 0 to 88; a higher score represents more severe symptoms. The inventory is broadly used to assess persistent TBI symptoms in veterans [21, 22]. It has high validity and consistency (α = 0.95) and reliability in assessing persistent TBI symptoms in veterans [23], as well as good test-retest values [24]. Translation and adaptation into Ukrainian were performed by Shvets et al. [25].
Posttraumatic Stress Disorder Checklist 5 (PCL-5) is a 20-item questionnaire for assessing PTSD symptoms with good internal consistency (α = 0.94), reliability (r = 0.82), convergent (rs = 0.74-0.85) and discriminant (rs = 0.31-0.60) validity, used to assess posttraumatic stress symptoms [26]. Total score ranges are from 0 to 80, with a greater score representing more severe symptoms. Translation and adaptation into Ukrainian were done by V. Bezsheiko [27].
Montreal Cognitive Assessment Scale (MoCA) is a valid, consistent (α = 0.83) and reliable scale used to assess cognitive functioning (attention, memory, executive functions, language, orientation), with total score ranges from 0 to 30; a greater score represents better functioning. Normal cognition is indicated by 26 points or higher [28]. Translation and adaptation into Ukrainian were done by Y. Trufanov [29].
Hospital Anxiety and Depression Scale (HADS) is a 14-item questionnaire used to assess symptoms of depression (HADS-D subscale, 7 questions) and anxiety (HADS-A subscale, 7 questions) [30]. It is a valid, consistent (α = 0.94) and reliable measure of anxiety and depression symptoms [30]. Each subscale score ranges from 0 to 21, with a greater score representing more severe symptoms of anxiety/depression respectively. Translation and adaptation into Ukrainian were done by Ahaiev et al. [31].
Positive and Negative Affect Scale (PANAS) is a 20-item questionnaire used to assess positive (PANAS+ subscale, 10 questions) and negative (PANAS– subscale, 10 questions) affect [32]. The minimum possible total score for each subscale is 10 points, the maximum is 50 points, a greater score represents higher positive or negative affect (respectively to subscale). PANAS is recommended as a scale with which to measure affect as a resilience factor [14]. PANAS subscales have good validity (α = 0.89 for positive affect subscale, α = 0.88 for negative affect subscale) [33]. Subtests scores range from 10 to 50 [34]. Translation and adaptation into Ukrainian were done by Klimanska and Haletska [33].
Chaban Quality of Life Scale (CQLS) is a 10-item questionnaire used to assess satisfaction with different aspects of life [35], with total score ranges from 0 to 100; a greater score indicates better overall quality of life. The scale has good internal consistency (α = 0.905), reliability (r = 0.923), convergent and discriminant validity [35]. The language of original scale is Ukrainian.
Data analysis
Data was stored in licensed Microsoft Excel 365 and analysis was conducted in the free statistical software EZR on R commander v 1.54. Continuous data were reported as mean ± standard deviation (SD) or median with interquartile range (as appropriate), proportions were provided as number of cases and frequency (n, %). The normality of data distribution was assessed with the Shapiro-Wilk test. The Pearson correlation coefficient was used to perform a correlation analysis. Pearson’s χ2 test was used to compare the frequency distributions between different demographic groups. Student’s t-test was used to compare the groups on continuous results. The Mann-Whitney test was used in cases where the data failed to meet the criteria for using the t-test or in those with small samples (n ≤ 5, [36, 37]).
Correlation analysis was performed using Pearson and Spearman tests as appropriate. The level of significance was set at p < 0.05, and the confidence level was set at 95%.
Following the task of distinguishing the effects of different variables [38] multivariate linear regression analyses was performed, including standardized betas to determine the weight coefficients of resilience factors. Multiple linear regression is more flexible than t test and correlational analysis, allowing the modeling of more than 1 independent variable [38]. 6 models were run, starting with the model (Model 1), including only socio-demographical independent variables and resilience as the dependent variable. Then stepwise forward selection was used to include a block of clinical variables (Model 2), and a block of cognitive and affective variables (Model 3). The full model included all socio-demographic, clinical, cognitive and emotional factors as independent variables (Model 3). Then stepwise backward selection was used to exclude the socio-demographic variables (Model 4) and then clinical variables (Model 5) to see the weight of cognitive and affective factors. The order of inclusion and exclusion were based on the hypotheses that: 1) significant additional variance of resilience may be explained by clinical, cognitive, and affective variables, 2) resilience in veterans with TBI can be explained mostly by clinical, cognitive, and affective variables, rather than by socio-demographic ones, and 3) a set of cognitive and affective variables still explain most of the variability in resilience even after the exclusion of clinical variables from the analysis. To assess the goodness of fit of the model adjusted R2 and residual plots analysis were used [38]. The Breusch-Pagan test was used to check for heteroskedasticity in a linear regression model. For data visualization we used the matplotlib and seaborn modules for the Python programming language.
RESULTS
A total of 146 veterans with persistent TBI symptoms participated in the study, 141 were male (96.58%) and 5 female (3.42%). 83 veterans were married (56.85%) and 63 were single (43.15%). 83 (56.85%) had a history of concussion while 63 (43.15%) had a history of mild cerebral contusion. The descriptive statistics of the variables investigated are presented in Table 1.
Table 1
Socio-demographic, clinical, cognitive, and affective variables in veterans with TBI (N = 146)
[i] CD-RISC – Connor-Davidson Resilience Scale, NSI + PCL – sum of Neurobehavioral Symptom Inventory and Posttraumatic Stress Disorder Checklist scores, MoCA – Montreal Cognitive Assessment Scale, HADS – sum of Hospital Anxiety and Depression Scale subscales, PANAS – Positive and Negative Affect Scale (“+” – positive affect subscale, “–“ – negative affect subscale), CQLS – Chaban Quality of Life Scale.
Correlation between resilience and socio-demographic variables
The study sample was characterized by a normal distribution of indicators on the CD-RISC scale (W = 0.993, p = 0.772). The mean value in the study population was 62.17 ± 13.08. The minimum value was 22 points, the maximum 100 points. No difference was found between males and females in the CD-RISC score (p = 0.317).
CD-RISC total scores in married veterans visually tended to be slightly higher (meaning better resilience) than in single veterans. However, no difference between the groups was found (t = 1.38, p = 0.168). CD-RISC total scores were 63.48 ± 13.93 for married veterans and 60.46 ± 11.77 for single ones. The distribution was normal for the sample of married veterans (W = 0.976, p = 0.129) and for single ones (W = 0.979, p = 0.383).
No correlation was found between age and CD-RISC total score (r = 0.097, p = 0.24) as well as between education and CD-RISC total score (r = 0.003, p = 0.965), indicating the absence of relationship between resilience and these variables.
The average value on the CD-RISC total scale for veterans with concussion was 60.83 ± 13.05, and for veterans with mild cerebral contusion was 63.95 ± 13.04. In both groups, depending on the clinical type of mild TBI, resilience had a normal distribution (p > 0.05). It was also found that there was no statistically significant difference between the CD-RISC averages based on the TBI clinical type (t = –1.43, p = 0.154).
No significant relationships between resilience and deployment duration (r = –0.051, p = 0.541), resilience and number of TBI events (r = 0.040, p = 0.628), resilience and time since last TBI (r = 0.013, p = 0.875) were found.
Correlation with clinical, cognitive, and affective variables
It was found that resilience correlated with different clinical, cognitive, and affective variables. Correlation plots are presented in Figure I.
Figure I
Scatter plots, representing correlations between resilience and clinical, cognitive, affective variables
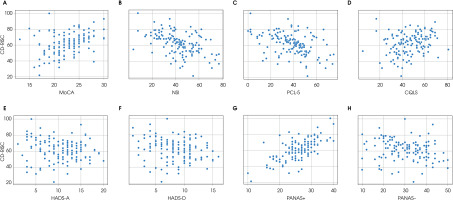
Significant negative correlations were found between resilience and neurobehavioral symptoms of TBI (r = –0.4, p < 0.001), and resilience and post-traumatic stress symptoms (r = –0.44, p < 0.001). Significant positive correlations between resilience and cognitive performance (r = 0.41, p < 0.001), resilience and positive affect (r = 0.66, p < 0.001), resilience and quality of life (r = 0.28, p < 0.001) were found. No significant correlations were found between resilience and anxiety (r = –0.11, p = 0.177), resilience and depression (r = –0.23, p = 0.099), and resilience and negative affect (r = –0.17, p = 0.053).
Multivariate regression analysis
After the correlation analysis a multivariate linear regression was performed, with resilience as the dependent variable and estimated value of different predictors. Prior to the regression analysis we analyzed a correlation matrix to exclude the possible multicollinearity effect. There was a high correlation between the NSI and PCL scores (r = 0.7, p < 0.001), and between HADS subscores (r = 0.567, p < 0.001). Also, the variance inflation factor (VIF) of NSI and PCL total scores in the regression model with all variables was 2.99 and 2.65 respectively, indicating that the multicollinearity effect is present. To reduce the multiple correlations effect, the scores of the NSI and PCL were combined into one variable NSI + PCL (the sum of their scores), and HADS subscores’ totals were combined into one variable HADS.
When the stepwise forward regression was started with adding only a block of socio-demographic variables, it was found that no statistically significant predictors were in the model and that Model 1 overall could not significantly explain variance in resilience (p = 0.561). After adding a block of clinical variables to another model (Model 2) it was found that more severe neurobehavioral symptoms were associated with less effective resilience (β = –0.456, t = –5.68, p < 0.001), and that better quality of life was associated with better resilience (β = 0.172, t = 2.12, p < 0.001); also that the overall model became statistically significant in explaining resilience in predicting ≈28% of its variance (Adj. R2 = 0.279, p < 0.001). However, adding a block of cognitive and emotional variables to the model (Model 3) increased the power of the model to predict resilience variance up to 57%. The regression analysis indicated that, when all variables were included in the model (Model 3), the only significant predictor among socio-demographic variables was length of deployment – the more time a combatant spent in the warfare zone, the less effective resilience he/she had even years after the trauma (β = –0.145, t = –2.22, p < 0.05). More severe neurobehavioral and posttraumatic symptoms were also predictors of bad resilience (β = –0.166, t = –2.00, p < 0.05), while better quality of life was connected to better resilience (β = 0.140, t = 2.01, p < 0.05). Good cognitive performance related to better resilience (β = 0.182, t = 2.57, p < 0.05), as well as positive affect (β = 0.554, t = 7.39, p < 0.001). Cognitive functioning, positive affect, and the sum of neurobehavioral and posttraumatic symptoms were the most valuable predictors in the Model 3. Results of the stepwise forward regression are presented in Table 2.
Table 2
A summary of stepwise forward regression analysis for sample characteristics as predictors of resilience (CD-RISC), n = 146
Model 1: F(8, 137) = 0.850, p = 0.561, R = 0.217, R2 = 0.047, Adj. R2 = –0.008, VIF < 2, AIC = 1177.21.
Model 2: F(11, 134) = 6.092, p < 0.001, R = 0.577, R2 = 0.333, Adj. R2 = 0.279, VIF < 2, AIC = 1131.075.
Model 3: F(14, 109) = 12.661, p < 0.001, R = 0.787, R2 = 0.619, Adj. R2 = 0.570, VIF < 2, AIC = 900.272.
B – regression coefficient, β – standardized regression coefficient, SE – standard error, t – Student’s test value, CD-RISC – Connor-Davidson Resilience Scale, NSI + PCL – sum of Neurobehavioral Symptom Inventory and Posttraumatic Stress Disorder Checklist scores, MoCA – Montreal Cognitive Assessment Scale, HADS – sum of Hospital Anxiety and Depression Scale subscales, PANAS – Positive and Negative Affect Scale (“+” – positive affect subscale, “–“ – negative affect subscale), CQLS – Chaban Quality of Life Scale.
After excluding all socio-demographic variables (Model 4) from the full model, all clinical variables became non-significant, while cognition (β = 0.242, p < 0.001) and positive affect (β = 0.511, p < 0.001) were still significantly predicting higher resilience. In the final model (Model 5), after exclusion of all clinical variables, better cognitive performance and positive affect were still significant predictors of resilience (β = 0.316, p < 0.001 and β = 0.565, p < 0.001, respectively). It is notable that excluding socio-demographic and clinical variables from the model did not significantly reduce the R2. The variance inflation factor (VIF) for all variables in all 6 models was < 2, indicating the absence of multicollinearity. Results of the stepwise backward regression analysis are presented in Table 3.
Table 3
A summary of stepwise backward regression analysis for sample characteristics as predictors of resilience (CD-RISC), n = 146
Model 1: F(14, 109) = 12.661, p < 0.001, R = 0.787, R2 = 0.619, Adj. R2 = 0.570, VIF < 2, AIC = 900.272.
Model 2: F(6, 117) = 24.241, p < 0.001, R = 0.744, R2 = 0.554, Adj. R2 = 0.531, VIF < 2, AIC = 903.821.
Model 3: F(3, 120) = 44.125, p < 0.001, R = 0.724, R2 = 0.525, Adj. R2 = 0.513, VIF < 2, AIC = 905.813.
B – regression coefficient, β – standardized regression coefficient, SE – standard error, t – Student’s test value, CD-RISC – Connor-Davidson Resilience Scale, NSI + PCL – sum of Neurobehavioral Symptom Inventory and Posttraumatic Stress Disorder Checklist scores, MoCA – Montreal Cognitive Assessment Scale, HADS – sum of Hospital Anxiety and Depression Scale subscales, PANAS – Positive and Negative Affect Scale (“+” – positive affect subscale, “–“ – negative affect subscale), CQLS – Chaban Quality of Life Scale.
All models were compared by Akaike Information Criterion (AIC) score and it was found that adding cognitive and emotional variables into a model significantly reduced AIC, indicating better model fitting; however, excluding socio-demographical and clinical variables didn’t significantly increase AIC, meaning that Model 3, Model 4 and Model 5 are quite equally good in prediction for this sample. Therefore, for large sample size model with large amounts of predictors is a better choice, while for small sample sizes (as in this study) models with main clinical, cognitive and emotional variables or even models with only cognitive and emotional variables may be a better choice for building predictions to avoid overfitting. The highest F-statistics were in the last model.
Considering the high value of cognitive and affective variables in predicting resilience, we analyzed residuals in the final model (Model 5) to evaluate the goodness of a fitted model. Residual standard error for the final model was 9.112. Residual plots are presented at Figure II.
As can be seen from residual vs fitted plot, the residual data are equally distributed around the 0 with no outliers, meeting the assumption of equal residual variances and suggesting that the linear relationship is a reasonable assumption. As can be seen from quantile-quantile (Q-Q) plot, there is some slight deviation from the straight line in the right histogram tail; however, an additional Shapiro-Wilk test on residuals indicated a normal distribution of data (p > 0.05). From scale-location plot it can be seen that residuals appear to be randomly spread along the ranges of predictors, assuming homoscedasticity. The Breusch-Pagan test did not produce sufficient evidence for us to say that heteroscedasticity is present (p > 0.05) as well, thus we can assume homoscedasticity in the model. Analysis of leverage have shown that all cases are well inside the Cook’s lines and there are no influential cases. Overall, all diagnostics indicated that the model works well for the data from the study.
DISCUSSION
The current study examined socio-demographic, clinical, cognitive, and affective correlates of resilience in veterans with TBI. Correlational analyses showed that resilience in this group was related to positive affect and, to a lesser extent, it correlated with posttraumatic stress, cognitive performance, neurobehavioral symptoms, and quality of life. No significant relationships were found between resilience and such variables as gender, age, education, or marital status or characteristics of head trauma (clinical type, number of events and time since last event), anxiety, depression, and negative affect.
The regression analysis revealed that positive affect and cognitive performance are the most valuable predictors contributing most of the variance to resilience. Including these variables in the regression model more than doubles its prognostic capacity, while excluding all other variables except cognitive and affective did not significantly reduce the predictive power of the regression model.
Our results also complement those of other recent studies. A previous study of how resilience relates to socio-demographic variables in non-veteran TBI sample showed that age, gender, and marital status have no correlation with resilience; education, however, does [39]. We found no relationship between education and resilience in the study. We explain this either by the diminishing role of education in resilience years after the injury, or by the lesser education impact to resilience of veterans with TBI. Considering that in non-veteran and non-TBI samples resilience has correlated with different sociodemographic variables like age, marital status, and employment status [40], our results allow us to lean towards the first hypothesis, and subjective clinical indicators become much more correlated with resilience than sociodemographic ones. We found no prior studies that mentioned such a shift.
A recent study of US veterans indicated that low resilience is associated with poor neurobehavioral functioning even 10 years after injury [8]. It is also known that in veterans with TBI symptoms of posttraumatic stress and head injury have a negative impact on each other, making the prognosis worse [41]. Strong correlations between these symptoms in our study also confirm these findings. Less effective resilience is associated with greater severity of post-traumatic stress; therefore, its cultivation it is important in overcoming trauma [42]. It was argued that beyond the simple reduction of post-traumatic stress symptoms, due to their mediating effect resilience-based psychotherapeutic interventions may help to promote post-traumatic growth [42]. Considering resilience as a dynamic process related to recovery, it seems to be crucial to cultivate and enhance it to reduce TBI and post-traumatic stress symptoms.
However, we found that neurobehavioral symptoms and posttraumatic stress were valuable predictors of resilience before adding to the model the cognitive and affective variables. After doing so, the value of neurobehavioral and posttraumatic stress symptoms significantly reduced. This indicates that the characteristics of both clinical and brain traumas may be not the variables that most contribute to whether the person will overcome adversity or not. Some authors also argue that resilience has a robust influence on neurobehavioral functioning after head trauma [8]. Our analysis partially supports those findings, allowing us to suggest that neurobehavioral symptoms are more influenced by psychological resilience than resilience is influenced by neurobehavioral symptoms.
If good cognitive performance, positive emotions, and effective stress management are valuable for good resilience it is possible that, by targeting them in interventions, we can promote resilience. Horn & Feder theorized that enhancing cognitive flexibility and emotional regulation, and cultivating positive emotions may help to reinforce resilient functioning and promote post-traumatic growth [43]. Some studies in other populations have shown that this theory may be valid. McDaniel et al. showed a linear positive relationship between resilience and cognitive functioning in an older, non-TBI veteran sample [44]. A strong relationship between resilience and positive affect was also mentioned previously in studies with non-clinical samples [45]. However, there have been no studies supporting this idea regarding the population of veterans with TBI, particularly years after injury. We found evidence that cognitive and affective factors can be more important for resilience than clinical and sociodemographic ones, which has not been previously reported.
Therefore, we can say about the empirical evidence that cognitive and affective protective factors are highly important for good resilience in veterans years after TBI. Our study provides some evidence that in a sample of veterans with TBI cognitive and affective factors are more strongly associated with resilience than clinical or sociodemographic factors. These findings fully support new theoretical models of resilience that describe cognitive and affective processes as even more crucial than constitutional or clinical factors for overcoming TBI-related adversities [10, 11]. A recent US National Health and Resilience in Veterans Study underscored the need to develop intervention strategies that target modifiable resilience factors in veterans, yet it was concentrated mostly on personality and social characteristics related to resilience [46]. While resilience was previously considered to be a personality trait, or as a process mostly reliant on personality and social factors, the most recent approaches conceptualize resilience as a multidimensional, dynamic, and variable process. Considering cognitive and affective processes as resilience factors and/or components, we can make a shift from trait-related studies and interventions to discovering dynamic and truly modifiable resilience characteristics.
This may be valuable for the rehabilitation of veterans with TBI. Extrapolating these ideas to the context of military brain injury, we can see it as a way not only to reduce or compensate for trauma symptoms, but to help veterans take some valuable insights from this experience and promote further personal development. The concept of posttraumatic growth in this context then takes on a completely different meaning.
The findings presented need to be interpreted in the context of some limitations: 1) all the cross-sectional study limitations, like difficulty to definitely interpret relationships identified and susceptibility to biases; 2) the study sample underrepresented the female veteran population, which could possibly have skewed the results; 3) no data regarding severe TBI was presented; 4) the voluntary participation and involvement only of veterans who seek help in veteran healthcare facilities could have increased selection biases; 5) veterans who sought help only in Kyiv facilities participated, which could have led to an underrepresentation of other Ukraine regions; and 6) the absence of control sample (i.e., veterans with a recent head injury or veterans without TBI) could also have led to different findings.
CONCLUSIONS
Overall, we found that resilience in veterans with TBI has a strong correlation with clinical, cognitive, and affective variables like persistent neurobehavioral symptoms, posttraumatic stress, quality of life, cognitive functioning, and positive affect even years after their trauma. At the same time, no significant relationships with socio-demographic variables, brain injury characteristics or negative emotional states like anxiety and depression were found. Such variables as good cognitive performance and positive affect were found to be more valuable for effective resilience in veterans with persistent TBI symptoms (possibly as protective factors) than the clinical type of traumatic brain injuries, their number or the time passed since last trauma and that they can explain the great amount of variance in resilience even after excluding all other variables. Unlike many other factors, cognitive performance, raising positive emotions and effective stress management can be modified and enhanced. Targeting them in specific interventions will possibly cultivate resilience. Such resilience-oriented interventions have the potential not only to reduce or compensate for symptoms of TBI, but to build on this experience a foundation for further personal growth, and could therefore be valuable in the rehabilitation process.