Introduction
Colorectal cancer (CRC), a form of epithelial cancer arising from the glandular tissue of the colon and rectum, is the fourth most diagnosed cancer in the United States. Even though current epidemiological data show that the death rate for both men and women has been dropping for the past several decades, it remains the second most common cause of cancer-related deaths when the numbers of both sexes are combined [1]. In addition, the incidence of CRC in people under 50 years old has steadily increased, with symptomatic disease driving the need for further examinations and diagnosis at advanced stages, which is also associated with a poorer prognosis [2]. Thus, screening methods are needed now more than ever, with the most notable being routine colonoscopy, which allows direct visualization of suspicious lesions or polyps and tissue biopsy retrieval.
The evaluation of histopathological samples under microscopy remains the gold standard for the establishment of CRC diagnosis. This is done by examination of haematoxylin and eosin (H&E)-stained tissues under a microscope, examining an array of morphological microscopical tissue alterations, first and foremost the presence and depth of tissue invasion, and additional characteristics such as glandular architecture, cell polarity, the disappearance of glands, and the presence of desmoplastic reactions, to determine the deviation from normal tissue architecture and the presence of malignancy. The pathological report is therefore essential for the optimal treatment protocol selection and directly affects the patient’s length of survival. However, histopathological examination is a time-consuming process, which in combination with the worldwide pathologist shortage has led to an increased time for diagnosis, which contributes to delays in treatment. Moreover, this procedure is subjective by nature leading to inconsistent results between pathologists (inter-observer variability) [3, 4] as well as inconsistency in the same pathologist due to fatigue and medical burnout (intra-observer variability). To alleviate this process, computer-aided diagnosis (CAD) systems have recently been proposed to quantitively analyse digitalised counterparts of glass slides: whole slide imaging (WSI).
WSI, also referred to as virtual pathology, involves the creation of a very high-resolution digitalised analogue of the images obtained through the entire stained tissue as viewed under light microscopy. These images carry the inherent advantages of any computerised image such as magnification and free-hand navigation on any of its parts. Recent publications have proposed that WSI can be utilised for automated diagnostic tools that are capable of producing results highly similar to those of the human operator [5].
Many of the recently introduced CAD models have been used to assist pathologists in the evaluation of many tissue samples, such as lung, breast, and colon by minimizing inter- and intra-observer variability, and they have proven to be at least non-inferior in pathologic image classification [6]. The spectrum of trained algorithms ranges from conventional machine learning to the more advanced and widely used deep learning (DL) models, in the face of convolutional neural networks (CNNs). CNNs extract information from the digitalized RGB images, analyse them, and perform classification of the colorectal tissue sample to provide robust results and decrease the amount of time required for diagnosis.
Our current fund of knowledge lacks a clear understanding of the current state of the DL algorithms regarding CRC digital histology samples and whether there is enough data to support their implementation in the current evidence-based clinical practice as well as a systematic report of under-utilised capabilities of such models. The aim of this systematic review is to advance our understanding of these modern techniques, specifically examining their diagnostic usage in binary malignant detection and colorectal tissue classification.
Material and methods
Search strategy and study eligibility criteria
This systematic review was performed according to the updated Preferred Reporting Items for Systematic reviews and Meta-Analyses statement (PRISMA) and was submitted to PROSPERO for registration. The study period included PubMed literature searches from the Cochrane Library from October 2009 until 1 November 2022, with the following keywords for the electronic search: “convolutional neural networks”, “CNN”, “deep learning”, “colon cancer”, “malignant intestinal cancer”, “colorectal cancer”, “bowel cancer”, “biopsy”, “histology”, “microscopy images”, and “histopathology.” Systematic searches were conducted by 2 independent investigators who were blind to each other, and any discrepancies were resolved by consensus between them.
The systematic review was undertaken in accordance with the Preferred Reporting Items for Systematic Reviews and Meta-Analyses (PRISMA) guidelines [7]. Studies were eligible for inclusion provided they met the following criteria: presentation of the development of at least one machine learning, deep learning model for tissue classification or diagnosis, using a binary or multiple class outcome, with a training dataset that included histopathological colorectal tissue whole-slide images or segmentations of WSI (patches). Review articles, meta-analyses, or articles that presented the use of algorithms for analysis of images from endoscopic procedures or for a different outcome other than tissue classification and diagnosis were excluded. Institutional board review approval is not required for a study-level systematic review.
Study selection and data collection process
All results retrieved from the systematic search of electronic libraries were imported into Rayyan, and duplicates were manually removed. Titles, abstracts, and keywords of all the articles were screened by 2 independent reviewers, and irrelevant reports were removed. Full-text screening of the selected articles was performed by the same 2 reviewers. Each disagreement was resolved through discussion and consultation with the other authors.
A data extraction form was created to extract the study’s characteristics and model performance metrics. This form was evaluated for suitability in 2 randomly selected studies by all the study’s authors. After finalizing the form, 2 of the authors independently extracted the data from each study (Table I).
Table I
Deep learning models for diagnosis and classification of colorectal cancer
Author | Year | Country | Study type | Aim of model | Architecture | Task | Dataset used | Evaluation metrics |
---|---|---|---|---|---|---|---|---|
Kainz [34] | 2017 | Austria | Journal article | Classification of glands | Custom CNN | 4-class (benign, benign background, malignant, malignant background) | 2015 MICCAI Gland Segmentation Challenge, Training 85 Images (37 benign and 48 malignant) Testing 80 (37/43) | Detection results (MICCAI Glas): F1 score = (0.68 + 0.61)/2, DICE index (0.75 + 0.65)/2, Hausdorff (103.49 + 187.76)/2 |
Xu [35] | 2017 | China | Journal article | Gland Classification | Custom CNN | Binary (bening/malignant) | 2015 MICCAI Gland Segmentation Challenge Training 85 Images Testing 80 Images | Detection results (MICCAI Glas): F1 score (0.893 + 0.843)/2, DICE index (0.908 + 0.833)/2, Hausdorff (44.129 + 116.821)/2 |
Awan [36] | 2017 | UK | Journal article | Grading of CRC | UNET | (A) Binary (normal/cancer) (B) 3-class: normal/low grade/high grade | 38 WSIs, extracted 139 parts (71 normal, 33 low grade, 35 high grade) | (A) Binary ACC: 97% (B) 3-vlass ACC: 91% |
Chen [37] | 2017 | China | Journal article | Nuclei Classification | Custom CNN | Binary (benign/malignant) | 2015 MICCAI Gland Segmentation Challenge and 2015 MICCAI Nuclei Segmentation Challenge | Detection results (MICCAI Glas): F1 score = 0.887, DICE index 0.868 Hausdorff = 74.731 Segmentation results: D1 and D2 metrics from Challenge |
Xu [38] | 2017 | China | Journal article | Tissue Classification | Alexnet – SVM | (1) Binary (cancer/not cancer) | 2014 MICCAI Brain Tumour (incl. colorectal metastases) Digital Pathology Challenge | ACC: (1) Binary: 98% |
Haj-Hassan [39] | 2017 | France | Journal article | Tissue Classification | Custom CNN | Triple: benign hyperplasia (BH), intraepithelial neoplasia (IN), and carcinoma (Ca) | Biopsy images of 30 CRC patients | ACC: 99.17% |
Chaofeng | 2017 | China | Journal article | Tissue Classification | Custom Bilinear CNN | Eight types of tissue, namely tumour epithelium, simple stroma, complex stroma, immune cells, debris, normal mucosal glands, adipose tissue, and background (no tissue) | H&E-stained colorectal cancer histopathological image dataset from the University Medical Centre Mannheim | AUC value of 0.985 |
Van Eycke [40] | 2018 | Belgium | Journal article | Diagnosis | VGG | Binary (benign/malignant) | 2015 MICCAI Gland Segmentation Challenge Training 85 Images Testing 80 (37/43) | Detection results (MICCAI Glas): F1 score = (0.895 + 0.788)/2, DICE index (0.902 + 0.841)/2, Hausdorff (42.943 + 105.926)/2 |
Graham [41] | 2019 | UK | Journal article | Diagnosis | Custom Architecture MILD-net | Binary (benign/malignant) | (1) MICCAI Gland Segmentation Challenge (2) 38 WSIs, extracted 139 parts (71 normal, 33 low grade, 35 high grade) | (1) F1 score: (0.914 + 0.844)/2, Dice: (0.913 + 0.836)/2, Hausdorff (41.54 + 105.89)/2 (2) F1 score: 0.825, Dice: 0.875, Hausdorff: 160.14 |
Yoon [42] | 2019 | South Korea | Journal article | Diagnosis | Modified-VGG | Binary (benign/malignant) | Centre for CRC, National Cancer Centre, Korea, 57 WSIs, 10,280 patches | ACC: 93.48%, SP: 92.76%, SE: 95.1% |
Qaiser [9] | 2019 | UK | Journal article | Diagnosis | Custom CNN | Binary (benign/malignant) | (1) Warwick-UHCW 75 H/E WSIs (112,500 patches), (2) Warwick-Osaka 50 H/E WSIs (75,000 patches) | (A) PHP/CNN: F1 score 0.9243, Precision 0.9267 (B) PHP/CNN: F1 score 0.8273, Precision 0.8311 |
Sari [43] | 2019 | Turkey | Journal article | Grading of CRC | Feature Extraction from Deep Belief Network and classification employing linear SVM, Comparison with Alexnet, GoogleNet, Inceptionv3, and autoencoders | (1) 3-class: normal (N), Low Grade (LG), High Grade (HG) (2) 5-class: Normal, Low (1), Low (1–2), Low (2), High | (1) 3236 images 1001 N, 1703 LG, 532 (HG) (2) 1468 images | (1) mean ACC: 96.13 (2) mean ACC: 79.28 |
Rączkowski [44] | 2019 | Poland | Journal article | Tissue Classification | Custom CNN | (A) Binary: tumour/stroma (B) 8-class: tumour epithelium, simple stroma, complex stroma, immune cells, debris, normal mucosal glands, adipose tissue, background | 5000 patches | (1) AUC 0.998 ACC: 99.11 ±0.97% (2) AUC 0.995 ACC: 92.44 ±0.81% |
Sena [26] | 2019 | Italy | Journal article | Tissue Classification | Custom CNN | Normal mucosa, preneoplastic lesion, adenoma, cancer | 393 WSIs | ACC: 81.7 |
Xu [35] | 2020 | Canada | Journal article | Diagnosis | Custom CNN | Binary (benign/malignant) | St. Paul’s Hospital, 307 H/E images | ACC: 99.9% (normal slides), ACC: 94.8% (cancer slides) Independent dataset: median ACC: 88.1%, AUROC: 0.99 |
Song [45] | 2020 | China | Journal article | Diagnosis | Custom CNN | Binary (benign/malignant) | 579 slides | ACC: 90.4, AUC: 0.92 |
Shaban [18] | 2020 | UK | Journal article | Grading of CRC | Custom CNN | 3-Class: normal, low grade, high grade | 38 WSIs, extracted 139 parts (71 normal, 33 low grade, 35 high grade) | ACC: 95.70 |
Iizuka | 2020 | Japan | Journal article | Tissue Classification | (1) Inception v3, (2)RNN | 3-class: adenocarcinoma/adenoma/non-neoplastic | 4.036 WSIs | (1) AUC: (ADC: 0.967, adenoma: 0.99), (2) AUC: (ADC: 0.963, adenoma: 0.992) |
Masud [46] | 2021 | Saudi Arabia | Journal article | Diagnosis | Custom CNN | Binary (benign/malignant) | LC25000 dataset, James A. Haley Veterans’ Hospital, 5000 images of Colon ADC, 5000 images of Colon benign tissue | ACC: 96.33% F-measure score 96.38% for colon and lung cancer identification |
Wang [6] | 2021 | China | Journal article | Diagnosis | Modified Inception v3 | Binary (bening/malignant) | 14,234 CRC WSIs and 170.099 patches | ACC: 98.11%, AUC: 99.83%, SP: 99.22%, SE: 96.99% |
Gupta [14] | 2021 | Switzerland | Journal article | Diagnosis | (a) VGG, ResNet, Inception, and IR-v2 for transfer learning, (b) Five types of customized architectures based on Inception-ResNet-v | Binary (benign/malignant) | 215 H/E WSIs, 1.303.012 patches | (a) IR-v2 performed better than the others: AUC: 0.97, F-score: 0.97 (b) IR-v2 Type 5: AUC: 0.99, F-score: 0.99 |
Yu [10] | 2021 | China | Journal article | Diagnosis | SSL | Binary (benign/malignant) | 13.111 WSIs, 62,919 patches | Patch-level diagnosis AUC: 0.980 ±0.014 Patient-level diagnosis AUC: 0.974 ±0.013 |
Toğaçar [47] | 2021 | Turkey | Journal article | Diagnosis | YOLO-based DarkNet-19 | Binary (benign/malignant) | 10,000 images | Overall ACC: 99.69% |
Terradillos [48] | 2021 | Spain | Journal article | Diagnosis | Custom CNN based on Xception | Binary (benign/malignant) | 14,712 images | SE: 0.8228, SP: 0.9114 |
Paladini [28] | 2021 | Italy | Journal article | Tissue Classification | 2 × Ensemble approach ResNet-101, ResNeXt-50, Inception-v3 and DensNet-161. (1) Mean-Ensemble-CNN approach, the predicted class of each image is assigned using the average of the predicted probabilities of 4 trained models. (2) In the NN-Ensemble-CNN approach, the deep features corresponding to the last FC layer are extracted from the 4 trained models | 7-class, 8-class, respectively | WIS-Kather-CRC-2016 Database (5000 CRC images) and CRC-TP Database (280,000 CRC images) | Kather-CRC-2016 Database: Mean-Ensemble-CNN mean ACC: 96.16% NN-Ensemble-CNN mean ACC: 96.14% CRC-TP Database: Mean-Ensemble-CNN ACC: 86.97% Mean-Ensemble-CNN F1-Score: 86.99% NN-Ensemble-CNN ACC: 87.26% NN-Ensemble-CNN F1-Score: 87.27% |
Ben Hamida [24] | 2021 | France | Journal article | Tissue Classification | (1) Comparison of 4 different architectures Alexnet, VGG-16, ResNet, DenseNet, Inceptionv3, with transfer learning strategy (2) Comparison of SegNet and U-Net for semantic Segmentation | (A) 8-class: tumour, stroma, tissue, necrosis, immune, fat, background, trash (B) Binary (tumour/no-tumour) | (1) AiCOLO (396 H/E slides), (2) NCT Biobank, University Medical Centre Mannheim (100,000 H/E patches), (3) CRC-5000 dataset (5000 images), (4) Warwick (16 H/E) | (1) ResNet On AiCOLO-8: overall ACC: 96.98% On CRC-5000: ACC: 96.77% On NCT-CRC-HE-100: ACC: 99.76% On merged: ACC: 99.98% (2) On AiCOLO-2 UNet: ACC: 76.18%, SegNet: ACC: 81.22% |
Zhou [49] | 2021 | China | Journal article | Tissue Classification | Custom CNN with Res-Net | Binary (benign/malignant) | TCGA 1346 H/E WSIs | ACC: 0.946 Precision: 0.9636 Recall: 0.9815 F1 score: 0.9725 |
Riasatian [30] | 2021 | Canada | Journal article | Tissue Classification | Custom CNN based on DenseNEt | 8-class: tumour epithelium, simple stroma, complex stroma, immune cells, debris, normal mucosal glands, adipose tissue, background | The Cancer Genome Atlas’ 5000 patches | ACC: 96.38% (KN-I) and 96.80% (KN-IV) |
Tsuneki [25] | 2021 | Switzerland | Journal article | Tissue Classification | EfficientNetB1 model | 4-class: poorly differentiated ADC, well-to-moderately differentiated ADC, adenoma, non-neoplastic) | 1799 H/E WSIs | AUC: 0.95 |
Jiao [29] | 2021 | China | Journal article | Tissue Classification | DELR based | 8-class: normal mucosa, adipose, debris, lymphocytes, mucus, smooth muscle, stroma, tumour epithelium | 180,082 patches | AUC: > 0.95 |
Kim [27] | 2021 | South Korea | Journal article | Tissue Classification | Custom CNN | 5-class: ADC, high-grade adenoma with dysplasia, low-grade adenoma with dysplasia, carcinoid, hyperplastic polyp | 390 WSIs | ACC: 0.957 ±0.025 Jac: 0.690 ±0.174 Dice: 0.804 ±0.125 |
Yan [19] | 2022 | China | Journal article | CRC Grading | Custom Divide-and-Attention Network (DANet) + Majority Voting | 3-class (normal, low high grade) | 15,303 patches as proposed by Awan et al. | ACC: 95.3%, AUC: 0.94 |
Dabass [20] | 2022 | India | Journal article | CRC Grading | Custom CNN based on Enhanced Convolutional Learning Modules (ECLMs), multi-level Attention Learning Module (ALM), and Transitional Modules (TMs) | 3-class (normal, low high grade) | Gland Segmentation challenge (GlaS), Lung Colon(LC)-25000, Kather_Colorectal_Cancer_Texture_Images (Kather-5k), NCT_HE_CRC_100K(NCT-100k) and a private dataset Hospital Colon (HosC) | GlaS (Accuracy (97.5%), Precision (97.67%), F1-Score (97.67%), and Recall (97.67%)), LC-25000 (Accuracy (100%), Precision (100%), F1-Score (100%), and Recall (100%)), and HosC (Accuracy (99.45%), Precision (100%), F1-Score (99.65%), and Recall (99.31%)), and while for the tissue structure classification, it achieves results for Kather-5k (Accuracy (98.83%), Precision (98.86%), F1-Score (98.85%), and Recall (98.85%)) and NCT-100k (Accuracy (97.7%), Precision (97.69%), F1-Score (97.71%), and Recall (97.73%)) |
Kassani [13] | 2022 | USA | Journal article | Diagnosis | Comparison of ResNet, MobileNet, VGG, Inceptionv3, InceptionResnetv2, ResNeXt, SE-ResNet, SE-ResNeXt | Binary (Healthy/Cancer) | DigestPath, 250 H/E WSIs, 1.746 patches | Dice: 82.74% ACC: 87.07% F1 score: 82.79% |
Shen [50] | 2022 | China | Journal article | Diagnosis | Custom CNN based on DenseNEt | 3-class: loose non-tumour tissue, dense non-tumour tissue, gastrointestinal cancer tissues | 1063 TCGA samples | DP-FTD: AUC 0.779, DCRF-FTD: AUC: 0.786 |
Chehade [15] | 2022 | France | Journal a rticle | Diagnosis | XGBoost, SVM, RF, LDA, MLP and LightGBM | Binary (benign/malignant) for CRC | LC25000 dataset | ACC of 99% and a F1-score of 98.8% for XGBoost |
Collins [16] | 2022 | Italy | Prospective study | Diagnosis | Custom CNN | 3-class (normal, T1-2, T3-4) | Images from 34 patients | 15-fold cross-validation (Se: 87% and Sp: 90%, respectively), ROC-AUC: 0.95. T1-2 group Se: 89%, Sp: 90%, T3-4 group, Se: 81%, Sp: 93% |
Ho [12] | 2022 | Singapore | Journal article | Diagnosis | Faster-RCC Architecture | Binary (low risk/high risk) | 66,191 image tiles extracted from 39 WSIs, Evaluation 150 WSI biopsies | AUC of 91.7% |
Reis [51] | 2022 | Turkey | Journal article | Nuclei Classification | Custom method (DenseNet169 + SVM, DenseNet169 + GRU) | 10-class | 10-class MedCLNet visual dataset consisting of the NCT-CRC-HE-100 K dataset, LC25000 dataset, and GlaS dataset | 95% accuracy was obtained in the DenseNet169 model after pre-train |
Albashish | 2022 | Jordan | Journal article | Tissue Classification | (1) E-CNN (product rule), (2) E-CNN (majority voting) | 4-class and 7 class | Stoean (357 images) and Kather colorectal histology (5000 images) | (1) ACC: 97.20%, (2) 91.28% |
Li [31] | 2022 | Hong Jong | Journal article | Tissue Classification | Pretrained ImageNet | 9 class | 100,000 annotated H&E image patches | ACC: 98.4% |
The study of bias assessment was conducted using the Quality Assessment for Diagnostic Accuracy Studies (QUADAS-2) tool, to assess studies regarding diagnostic tests (Supplementary Table SI). QUADAS-2 is a highly validated tool, focusing on 4 domains: patient selection, index tests, reference standard, and flow and timing (Supplementary Table SII). Each domain is assessed on 2 levels ranked as low/high/unclear risk of bias and concerns regarding applicability. More information on the tool itself and the assessment process can be found in the corresponding reference [8].
Results
The systematic review searches recognised 309 articles for potential inclusion. After title and abstract screening, 69 were deemed eligible for full-text screening. Overall, 41 articles were considered for this systematic review in accordance with the inclusion criteria. Our systematic search of the literature is depicted in more detail in the PRISMA flowchart (Figure 1). All details regarding the study origins, and the number of included images can be viewed in Table I.
Figure 1
Study selection process according to Preferred Reporting Items for Systematic Reviews and Meta-Analyses (PRISMA) guidelines
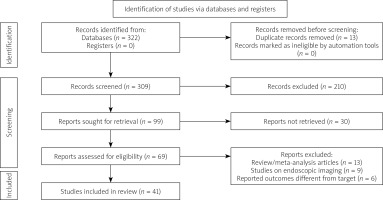
For evaluating the performance of a model, sometimes it is more useful to have a one-number summary than to examine both the sensitivity and the specificity. Performance metrics were evaluated wherever available, and the rest of the discussion was based on qualitative results from the literature, fulfilling the inclusion criteria. To compare the performance of the 2 sub-categories of models (customized vs. pre-trained) the Mann-Whitney U test for comparison of means was employed. One widely used metric is balanced accuracy (ACC). Since specificity and sensitivity are rates, it is more suitable to compute the harmonic average. In fact, the F1-score is the harmonic average of precision and recall, and it has been regarded as the preferred performance metric. It is worth mentioning that the size of the datasets ranged greatly from 38 to 14,234 WSIs (170,099 patches).
Binary outcomes (benign or malignant)
The simplest result for DL techniques is to return a binary outcome (yes or no) of whether the sample includes any suspicious parts for malignancy, because the answer to this question alters the therapeutic plan completely.
For this reason, to provide more robust results Qaiser et al. [9] tested 2 convolutional neural network (CNN) models while also using persistent homology profiles of topological features of WSIs, with the authors reporting the highest F1 score achieved to be 92% on a retrospectively obtained dataset. Furthermore, Yu et al. [10], using a database of 13,111 WSIs from 13 centres, constructed a semi-supervised learning algorithm (SSL), which performed equally to pathologists for CRC recognition.
A recent trend that is being adopted is transfer learning models. Transfer learning is a subclass of machine learning that implements knowledge used in an already existing model (pre-trained) in order to tackle a different but related task using a new model, with the main advantages being better performance and shorter training time. Utilising InceptionV3 as the basis, Xu et al. [11] trained a CNN model for screening with 99.9% accuracy on normal slides compared to a pathologist, which was pretrained on ImageNet – a well-known image dataset that follows a nodular organization of image groups that illustrate a word and its cognitive synonyms. ImageNet utilises the grouping of nouns into cognitive synonyms (nodes) formed by WordNet, created according to conceptual relationships between words, to categorize and organize into nodule images pertaining to or depicting these words. A different multicentre study that compared the performance of an AI pre-trained model with pathologists was presented by Wang et al. [6], using a large database (14,680 WSIs) with the reported AUC for AI being 99% vs. 97% of the pathologists. Another unique AI model was developed by Ho et al. [12], which was based on a faster-region-based CNN (faster-RCNN) with ResNet as a backbone, and which simultaneously segmented the glands from the WSIs into high-risk or low-risk while also classifying them into the following: benign glands, glands that are either characteristic for adenocarcinoma or high-grade dysplasia, low-grade dysplasia, blood vessels, necrosis, mucin, or inflammation. Despite the model’s high sensitivity (97.4%), the small dataset limits its generalization.
Direct comparison of the state-of-the-art pre-trained CNN feature extractors on different segmentation architectures was conducted by Kassani et al. [13], who underscored that shared DenseNet and LinkNet architecture is the one with the most potential, with reported accuracy of 87.07% and F1-score of 82.79%. In this domain, a study by Gupta et al. [14] compared the performance of many pre-trained techniques for discriminating the abnormal from normal patches obtained from digitalized images, with IR-v2 performing better than the rest without sacrificing time for diagnosis. Another comparative study, using the LC25000 dataset, which includes both lung and CRC images, tested 6 different pre-trained models and compared their performance. The results showed that the XGBoost model had a higher accuracy of 99% and an F1-score of 98.8% [15].
Following the current trends of machine learning research in histopathology, a team led by Collins et al. tried to extrapolate the pre-trained CNNs and utilise them to detect the presence of cancer-free margins in hyperspectral images (HIS) of surgical specimens. This study highlights a novel field of CNN training, providing results even faster than conventional pathology, and tissue classification techniques in the operative setting. This approach is effectively an extension towards not only characterizing a specimen as benign or malignant but also determining the spatial boundaries of the malignant tissue [16].
Higher-class tissue classification and grading
To further advance the protean characteristics of the cellular level images, models that report higher class outcomes have also been proposed for grading purposes (normal tissue, low grade of differentiation, high grade of differentiation) and even models that are designed to classify the tissue in up to 9 types, such as adipose, background, debris, lymphocytes, mucus, smooth muscle, normal colon mucosa, cancer-associated stroma, and colorectal adenocarcinoma epithelium.
To tackle the CRC grading task (normal, low, and high grade of dysplasia, according to the WHO histopathological classification) Awan et al., Shaban et al., and Yan et al. [17–19] developed custom CNN models with reported accuracy of 91%, 95.7%, and 95.3%. The latter proposed CNN was also based on majority-voting (MV) technique, and it could also be used in different tissues like breast cancer WSI samples, proving its superiority. One of the largest evaluations for a CNN model was presented by Dabass et al. [20], which comprised enhanced convolutional learning modules (ECLMs), a multi-level attention learning module (ALM), and transitional modules (TMs) and was tested on 4 diverse, publicly available datasets (Gland Segmentation challenge [GlaS], Lung Colon [LC]-25000, Kather_Colorectal_Cancer_Texture_Images (Kather-5k), and NCT_HE_CRC_100K [NCT-100k]) and one from their department (HosC). The reported F1-score for cancer gland classification was as follows: GlaS 97.67%, LC-25000 100%, and HosC 99.65%, while also for the tissue classification: Kather-5k 98.85% and NCT-100k 97.71%.
Following the trend of transfer learning, Malik et al. [21] were among the first to propose a pre-trained CNN model (InceptionV3) and tested its accuracy, which reached 87% for multiclassification outcomes. In certain instances, however, the reported accuracy of the models was characterized by high values of standard deviation, such as in the work of Popa et al. [22] with a reported standard deviation of 4%. This variability of the accuracy metric could compromise the stability of the model’s performance. To overcome this hindrance, Albashish et al. designed 2 models (E-CNNs) that ensemble the previously pretrained transfer learning models DenseNet, MobileNet, VGG16, and InceptionV3 to maximize the efficiency of feature extraction and classification tasks. The reported accuracy of the 2 models was 95.20% and 94.52%, respectively, with a standard deviation that was much lower, calculated at 1.7% when tested on the dataset of Stoean et al. [23] In a different comparative study, Ben Hamida et al. [24] trained a “from-scratch” CNN model using the AiCOLO-8 database along with pre-trained CNN state-of-the-art models (AlexNet, VGG, ResNet, DenseNet, Inception) and externally validated them in a different very large WSI dataset comparing their results. The ResNet model achieved the highest accuracy of 96.98%. Moreover, a study [25] highlighted the feasibility of encompassing the spectrum of the CRC into 4 stages (non-neoplastic, adenoma, well-to-moderately differentiated ADC, poorly differentiated ADC) using DL techniques with comparable performance results, such as the proposed models of Sena et al. and Kim et al. [26, 27]. Three studies evaluated the performance of custom 8-category tissue type classification techniques on CRC patches reporting similar results [28–30]. Of note, although the databases that were evaluated had a significant number of patches, they were different from each other. Lastly, Li et al. proposed that fine tuning the ImageNet-based neural networks with histopathological images could significantly enhance the prediction performance with segmentation of up to 9 tissue types (adipose, background, debris, lymphocytes, mucus, smooth muscle, normal colon mucosa, cancer-associated stroma, and colorectal adenocarcinoma epithelium), while also being used for the prediction of gene mutation and expression [31].
Customized vs. pre-trained models
A comparison of reported metrics between customized and pre-trained models did not return any significant differences. To compare the average reported metrics from reporting studies, we employed the Mann-Whitney U test for comparison of mean values. On average, the F1-score reported by custom CNNs was calculated at 0.88, as opposed to 0.93 from pre-trained models, a difference that was not of statistical significance (p = 0.423). Classification accuracy was also non-significantly different between customized networks and pre-trained models, with a reported average of 0.95 and 0.953, respectively (p = 0.9). Therefore, no significant differences in terms of performance, can be detected between pre-trained and customized neural networks currently reported in the literature. Pre-trained networks are usually built similarly to the UNET example, as reported by Awan et al. The network performs pixel-based classification tasks by importing a pixel of a histopathological image as an input, then outputting a corresponding pixel that represents the probability that the pixel of origin belongs to a glandular structure or not, therefore characterizing the presence of tumour, as well as being able to classify its histological grade. Customised models largely follow the structure of the LeNet model proposed in 1998 [32] and are composed of convolutional layers that lead their output into a function, which produces the pixel’s probability of belonging to a pre-specified class (usually benign/malignant if it is a binary classification algorithm).
Attention learning models in tissue classification
Due to its recent rise in popularity within classification tasks, we separately discuss the performance of attention learning within tissue classification in CRC. Within the included studies, there were 2 reports of attention modelling techniques employed in pathological image analysis [20, 33]. Dabass et al. (2022) present the inclusion of an attention learning module within their CNN architecture, as an enhancer deep learning module, tasked with allocating bias towards the most informative features of those already extracted by enhanced convolutional learning modules previously involved in the image analysis. The goal of the attention learning module is to tackle the challenge of varying sizes of important pathological regions in an image, and therefore enhance the model’s target refinement capacity. The biasing mechanism effectively re-directs computational resources towards the classification-specific tasks only. The result of incorporating the attention module was to counter the gradual decrease of spatial resolution of malignant feature maps. The team ran an analysis of the classification model before and after the inclusion of the attention module [20]. They demonstrated an increase in all prediction metrics in both binary and multi-level classification tasks for colorectal tumours. In binary grade classification, all metrics (accuracy, precision, sensitivity, and specificity) were estimated at a range of 99.31–100% for the testing dataset. It must also be noted that this study utilised slides from a completely different dataset to the testing set, rather than utilising images from the same dataset, which could falsely generate better model performance metrics. Multi-class classification of tumour structure was also augmented after the introduction of the attention module, increasing the F1-score to a total of 97.7% (from 93% prior to the inclusion of the attention module). Integrating attention modelling overcame the variability in tissue patterns by selectively enhancing the weights of specific structures of interest for the classification of a tissue specimen. The model’s performance metrics on an entirely separate dataset, originating from different patients, is evidence that adding attention modelling modules to a CNN can help overcome the interpersonal tissue variability that exists in clinical practice and burdens the human operator.
Another example of attention modelling in pathological images of CRC is the one developed by Yan et al. [33]. The ultimate goal of their classification model was to classify cellular nucleic structures from histological slides as belonging to malignant tissue or not, following image decomposition into nucleic and non-nucleic structures. The proposed architecture followed a “divide-and-attention” structure. The initial model splits the image data into 3 categories and performs feature extraction. At the end, the branches are re-fused, using global average pooling, to obtain a total of 5 feature vectors. Data from these vectors are funnelled into the attention learning module which selects the most representative tissue features and allows the model to focus on them. Although the team does not report results before and after the inclusion of the attention model layer, the overall reported accuracy for their model was 95.33% with an AUC of 0.94. It must be noted, however, that both the training and the testing image sets were derived from the same dataset [34–51].
Discussion
In this systematic review we analysed 41 studies focusing on the binary (normal, malignant) and multiclass categorization and grading of digital colorectal tissue pathology using state-of-the-art CNN classifiers. The reported classification outcomes and measures of effect differed among studies, while reaching impressive individually high numbers with a mean balanced accuracy of 95.8 ±3.8% (the highest reported being 99.69%) and mean F1-Score of 89.7 ±9.8% (the highest reported being 99%), with only a few studies also co-reporting sensitivity, specificity, precision, and recall. Of note, the efficiency of the models increased in accordance with the years. However, upon closer investigation of the individual studies, there is a lack of a standardized approach in reporting the results as well as the heterogeneity in the training datasets, which makes a direct objective comparison between the studies impossible. Another common characteristic of the included studies is an inherent weakness in image acquisition. Most datasets reached their final number of images from far fewer pathological slides which they rotated or refocused slightly in order to obtain a different picture. However, the likeliness between these images can contribute to better classification performance metrics.
One possible explanation for the inter-model variability of the tissue segmentation classes could be the reflection of the inter-observer variability of Western versus Eastern pathologists. In Western countries the presence of a cancerous lesion is confirmed by invasion beyond the submucosal tissue (also referred to as Vienna classification), while in the Eastern model the diagnosis is based on inner structural and nucleic abnormalities of the epithelium (also referred to as Japanese classification). Despite the research for which the CRC spectrum is encompassed in its entirety, a unified method has yet to be finalized [51, 52].
A comparison of handcrafted feature-based models versus automated deep learning models also showed the superiority of unsupervised training in classification models, rather than feature-based classification [53]. Another point raised by several authors, regarding the comparison of different classification models, is the quality of the initial annotation by the pathologist, which can influence solely the malignancy-containing slides [28, 48]. Furthermore, the direct comparison of different models and classification architectures is further hindered by the variability of the tissue itself. For instance, higher histological grades of colorectal cancer have been pointed out as being a challenge for the deep learning algorithms due to the presence of irregular and dense structures that are an impediment for the segmentation algorithms [54]. There is a lack of reporting of histological grades in many of the included studies and a complete absence of comparison of performance metrics between tissue grades. Therefore, the introduction of machine learning-based tissue classification in true clinical practice first requires the resolution of such issues.
Furthermore, even though it is evident that the performance of the included CNNs for CRC diagnosis is on par with the clinical pathologists [52, 55], many of the studies’ generalization potential was hindered by the study design and the relatively small and proprietary nature of training and validation datasets of many individual studies. For this reason, it is often recommended to externally cross-validate using publicly available, accepted, and large datasets such as the TCGA database, GIaS, LCK25000, etc. Furthermore, estimations of at least 10,000 WSIs are required to train a CNN model for histopathology tasks without even accounting for the variation in each of the WSIs due to the digitalization process [34–36, 56–58]. From the studies included in this review, only 3 were evaluated with databases in accordance with this estimation, while 8 tested their results in widely available large datasets. Thus, standardized evaluation of a model’s architecture and reporting is a necessary step towards its clinical implementation. A few studies mention an existing discrepancy between the automated classification results and the expert pathologist’s diagnosis. Scanning only select slides, and slides that happen to contain no abnormal architecture of carcinomas, (despite the existence of malignancy in the tissue in a deeper slide) are some of the dangers that are already described by authors [55]. As a result, it should be noted that the macroscopic appearance of a tissue specimen, the selection of scanned slides, and the overall distribution and number of processed images from the slides of a specimen are still issues that remain to be resolved, and they are quite possibly the parts where a human operator might be called on to support a machine learning algorithm.
A more unified approach in reporting the results was performed in the AI models that participated in the Gland Segmentation in Colon Histology Images (GlaS) challenge in 2015 and onwards [59]. The GIaS challenge was conducted by the 18th International Conference on Medical Image Computing and Computer-Assisted Intervention (MICCAI), in which the proposed models competed with objective measures of effect to achieve the optimal gland segmentation. The metrics of performance consisted of the F1-score, Dice Score (evaluating similarity of sample A vs. sample B), and Hausdorff distance (measure for comparing the end result with the segmentation result) [59].
When looking into possible differences between pre-trained models and custom neural networks, we found no differences in the reported numerical outcomes for accuracy, specificity, and sensitivity of classification. It must be noted, however, that looking into the model builds described, pre-trained and CNNs, are largely based on the same mechanisms and model layers.
A subsection of models used for tissue classification employed attention learning algorithms. Although these instances were scarce in the current literature on CRC pathological classification [20, 33], they provide some insights that could enhance the model outcomes already predicted by other CNNs. There is, however, some outstanding criticism regarding attention modelling. One issue that the experts mention is that attention models are fitted on top of usually pre-existing CNN backbone architectures [60]. Therefore, the question remains to be answered: to what extend do the attention models (and in turn, their performance) rely on the backbone architecture on which they are placed? Objective techniques for model assessment must be created if the quality of “learning” is to be properly assessed. In addition, recent technologies such as wireless sensor networks (WSN), the Internet of things (IoT), and the Internet of surgical things (IoST) contribute significantly to the development of smart health monitoring systems and applications for early diagnosis of non-contagious diseases such as cancers [61].
Lastly, it is worth mentioning that the spectrum of CRC histology and CNNs is ever evolving, and recent advances include many more areas of interest, other than structural alterations like tumour microenvironment, prognosis and survival, nucleic alterations like microsatellite instability, specific gene mutation prediction, and more. The emergence of potent DL techniques that harness the widely available data can enrich the cancer diagnosis field with the introduction of new research fields that could also provide invaluable information for the diagnostic process and aid the therapeutic plan.
Although most of the included studies are of a high standard, there are still a few underreported parameters that still need to be assessed before such innovations are introduced within everyday practice. The vast majority of existing studies fail to take into account significant histological findings that constitute oncological parameters, such as histological subtypes, stage of colorectal cancer, tumour grade, necrotic debris, and peritumoural necrosis. Additionally, there is a lack of research endeavours regarding the histological classification of harvested lymph nodes, and there is very little work on the identification of metastatic carcinomas. Future work should also include the exploration of correlations between patient factors and the performance of such models. It is highly likely that patients with early-stage tumours (e.g. in situ carcinomas) pose a higher classification challenge to DL models, due to more subtle differences with normal tissue. On the other end of the spectrum, higher-grade carcinomas and tissue specimens with extremely distorted architectures are a hurdle for image segmentation algorithms and feature extraction models. Future research steps should include the use of such models in the clinical environment rather than testing them in pre-determined datasets, as well as setting up randomized controlled trials for true comparison with expert pathologists. In the long run, more work is needed, to determine whether the use of such methods influences treatment choices, patient survival, and disease-free survival.
Conclusions
The performance of the currently available CNNDL models is at least non-inferior to conventional image-pattern recognition from pathologists, exhibiting impressive accuracy. However, owing to the small-scale datasets, variability of their training data, and lack of large-scale external validation, generalization of these results is not yet possible. In all likeliness we are at least a few years away from large-scale, systematic inclusion of AI-assisted pathological reviews of specimens. Additionally, we cannot expect the first implementation of such approaches to fully replace pathologists; AI-assisted screening will aid in the reduction of work hours, lessening the time-to-diagnosis period in the process. Few studies tackle the issue of external validation, further solidifying the need for future ones being compared using the same large datasets and thus paving the way for their implementation in the evidence-based healthcare system. In our study we can conclude not only that the current state-of-the-art algorithms are non-inferior to pathologists for image analysis and classification tasks, but also that their generalization potential is still limited due to their inherent uniqueness in their training and lack of widely accepted external validation datasets.